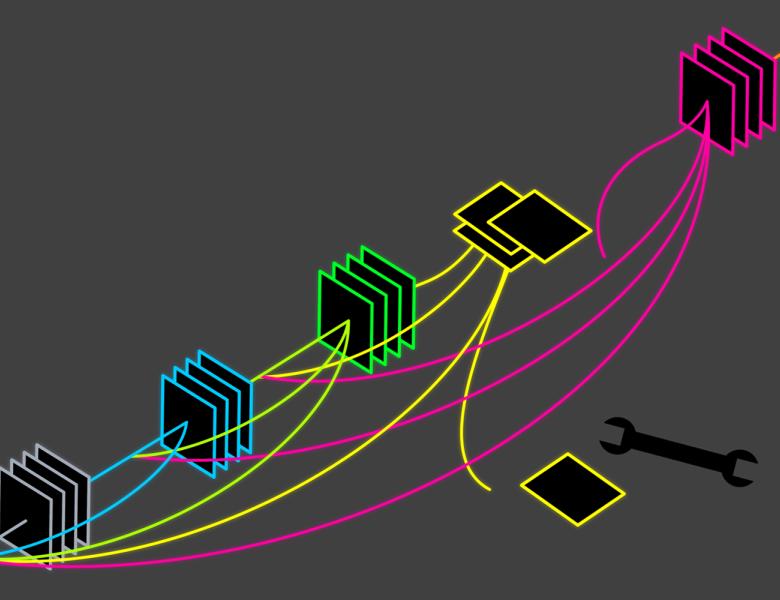
Why does deep learning work well for some applications and not for others? Do we need major architectural changes in deep learning to solve complex problems like natural language understanding and logic? Does memory and modular organization play an important role, and if so, how do we store complex concepts in memory? We will try to get a conceptual understanding of these questions by studying learning problems arising from synthetic mathematical function classes such as the learnability of polynomials, shallow teacher networks, and possible cryptographic hardness of learning deeper teacher networks. Finally we will present nascent ideas about how we should model memory and evolve a modular view of deep learning for higher level cognitive functions.
All scheduled dates:
Upcoming
No Upcoming activities yet