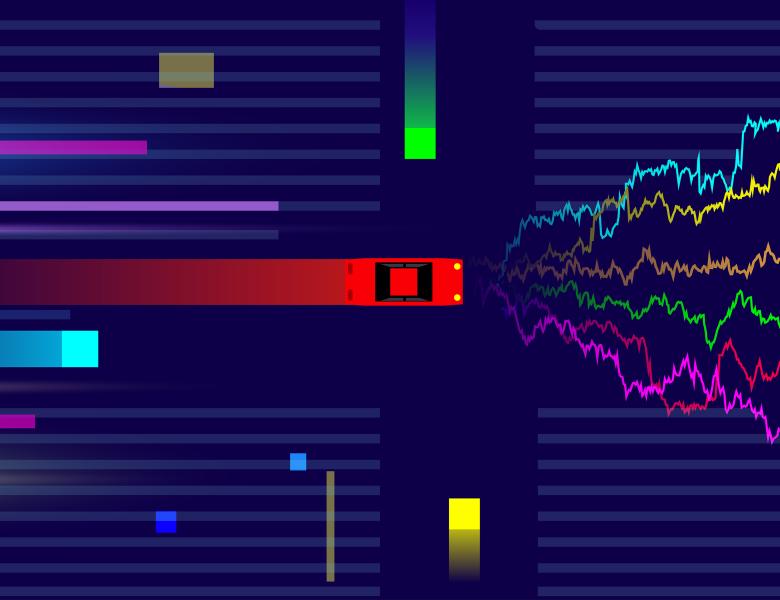
Title: Generalization Bounds via Online Learning
Abstract: Bounding the generalization error is one most fundamental problems in statistical learning theory. In this talk, I will explain a new
framework for deriving generalization bounds from the perspective of online learning. Specifically, we construct an online learning game called the Generalization Game, where an online learner is trying to compete with a fixed statistical learning algorithm in predicting the sequence of generalization gaps on a training set of i.i.d. data points. By construction of the game, the regret of the online learning can be shown equal to the generalization error of the statistical learning method, thus providing a way to prove generalization bounds via analyzing the regret of the online learner --- effectively reducing the statistical problem to a problem of numerical optimization. Our technique allows us to prove generalizations of the so-called "information-theoretic" generalization bounds of Russo & Zou (2016) and Xu & Raginsky (2017), as well as the classic PAC-Bayesian generalization bounds of McAllester, Catoni, Audibert, Langford, and Seeger.
(Based on joint work with Gabor Lugosi.)
All scheduled dates:
Upcoming
No Upcoming activities yet