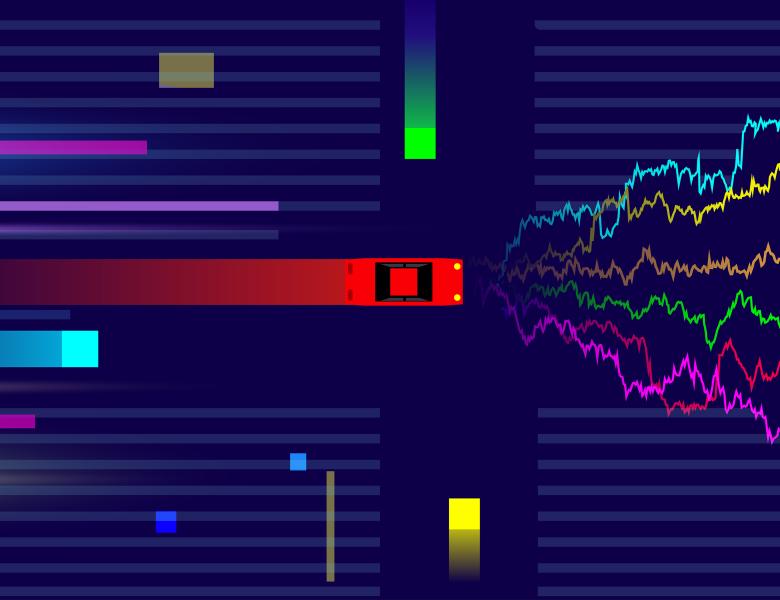
Title: A Tour of Instance Dependent Bounds for Interactive Learning
Abstract: In contrast to minimax, worst-case guarantees, an instance dependent bound scales with more fine grained properties of the stochastic problem instance. For example, the geometry of the features describing the arms for linear bandits, the diversity of the actions taken by policies (not just the number of) for contextual bandits, or the behavior of the state transition matrices for MDPs may all appear in instance dependent bounds. The objective is to not only characterize the intrinsic difficulty of these problem classes, but develop algorithms that adapt to the true difficulty of the problem. This talk will cover linear bandits, contextual bandits with general sets of policies, and MDPs in the tabular and linear function approximation settings.
All scheduled dates:
Upcoming
No Upcoming activities yet