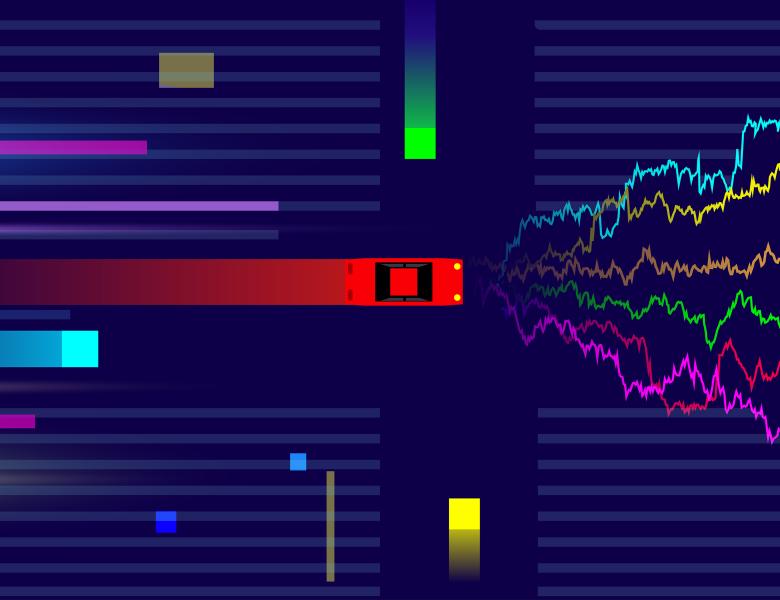
Title: Markovian Linear Stochastic Approximation: Bias and Extrapolation
Abstract: We consider linear stochastic approximation (LSA) with a constant stepsize and Markovian noise. We first establish weak convergence of the iterates to a limit and provide a non-asymptotic, geometric convergence rate. Furthermore, we provide a precise characterization of the asymptotic bias of this limit, establishing a Taylor-like, infinite series expansion thereof with respect to the stepsize. Consequently, the bias is proportional to the stepsize up to higher order terms of the stepsize. This result stands in sharp contrast for LSA with i.i.d. data, for which there is no bias. In fact, we show that the bias is roughly proportional to the mixing time of the Markovian noise.
While Polyak-Ruppert (tail) averaging reduces the variance, it does not affect the bias. The bias, however, can be reduced using Richardson-Romberg extrapolation. In particular, our infinite series expansion implies that extrapolation with any $m\ge2$ stepsizes eliminates the $m-1$ leading terms in the bias expansion and hence results in an exponentially smaller bias. As a by-product, we derive results for temporal difference (TD) learning algorithms with Markovian data and a constant stepsize.
All scheduled dates:
Upcoming
No Upcoming activities yet