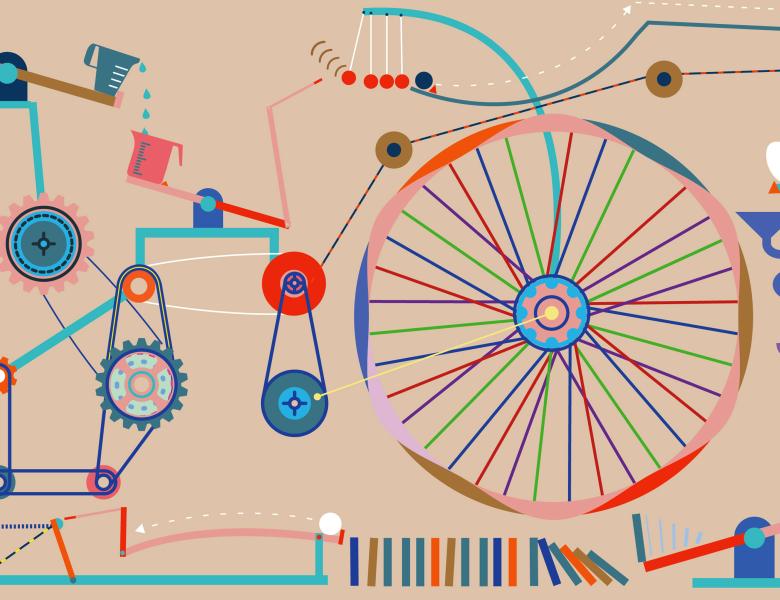
This meeting has been postponed and will be rescheduled sometime next week. Please check back later for updates.
Title: Predictability, stability, and causality with a case study to find genetic drivers of a heart disease
Abstract: "A.I. is like nuclear energy -- both promising and dangerous" -- Bill Gates, 2019.
Data Science is a pillar of A.I. and has driven most of recent cutting-edge discoveries in biomedical research and beyond. Human judgement calls are ubiquitous at every step of a data science life cycle, e.g., in choosing data cleaning methods, predictive algorithms and data perturbations. Such judgment calls are often responsible for the "dangers" of A.I. To maximally mitigate these dangers, we developed a framework based on three core principles: Predictability, Computability and Stability (PCS). The PCS framework unifies and expands on the best practices of machine learning and statistics. It consists of a workflow and documentation and is supported by our software package v-flow.
In this talk, we first illustrate the PCS framework through the development of iterative random forests (iRF) for predictable and stable non-linear interaction discovery (in collaboration with the Brown Lab at LBNL and Berkeley Statistics). In pursuit of genetic drivers of a heart disease called hypertrophic cardiomyopathy as a CZ Biohub project in collaboration with the Ashley Lab at Stanford Medical School and others, we use iRF and UK Biobank data to recommend gene-gene interaction targets for knock-off experiments. We then analyze the experimental data to show promising findings.
All scheduled dates:
Upcoming
No Upcoming activities yet