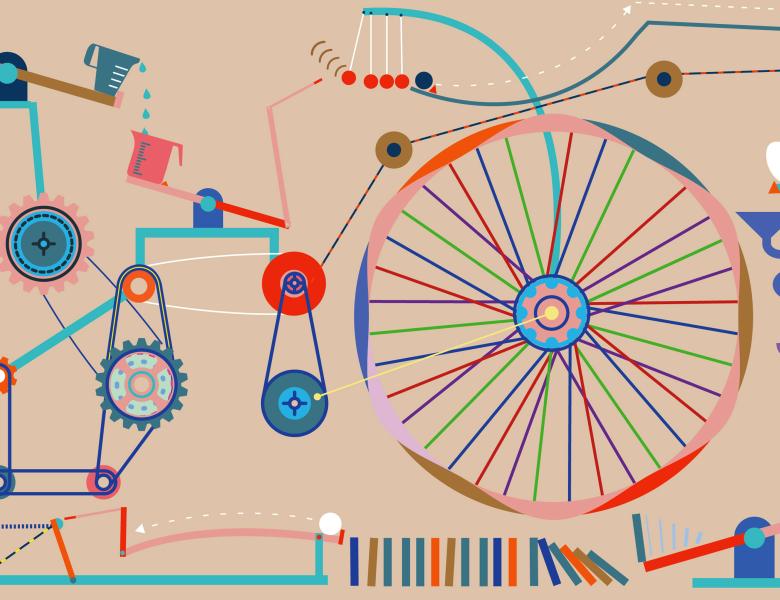
In healthcare settings, the goal is often to select the treatment that will maximize a specific patient's outcome; however, we often do not have sufficient randomized controlled trial data to reliably estimate the conditional treatment effect. Observational data on the other hand are usually plentiful but may be biased for effect estimation. In this talk, we discuss how we can use causal graphical models to combine observational and experimental data to improve causal effect estimation. Specifically, we discuss how to select optimal feature sets for post-intervention outcome prediction (a) when we know the causal graph and only have observational data, and (b) when we do not know the causal graph but have observational and limited experimental data.
All scheduled dates:
Upcoming
No Upcoming activities yet