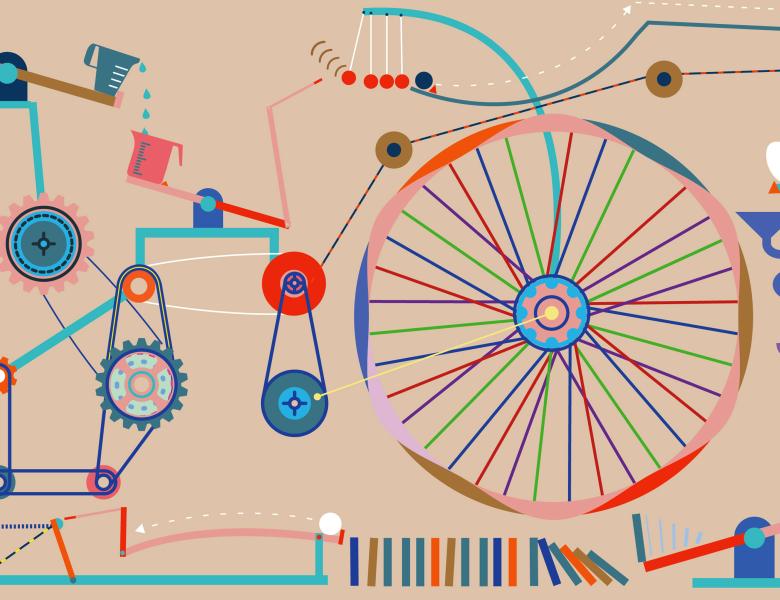
Abstract: Insofar as science aims at generalizable knowledge of dynamical systems, an important piece of scientific inference is the identification of classes of system over which dynamical features will generalize. Traditionally, this has been viewed in terms of abstraction -- first, similarities are noted among specific dynamical models and differences are then abstracted away in order to identify a class with shared dynamics. But by clarifying the causal structural features that members of such scientifically salient classes have in common, it is possible to detect class membership without first learning detailed dynamical models. In other words, it's possible to determine dynamical similarity without knowing the dynamics.
In this talk, I describe the use of dynamical symmetries -- interventions or manipulations of a system that commute with its time evolution -- to define dynamical kinds, and present a new metric of dynamical similarity that provides a natural measure of similarity among these kinds. I show that this metric tracks how much systems differ in their effective order, degree of nonlinearity or chaos, and the distribution of stochastic sources of behavior. I also outline how this metric can be accurately estimated from data, and sketch three major areas of application: data-driven clustering of dynamically similar systems, structural change detection, and causally sensitive model validation.
All scheduled dates:
Upcoming
No Upcoming activities yet