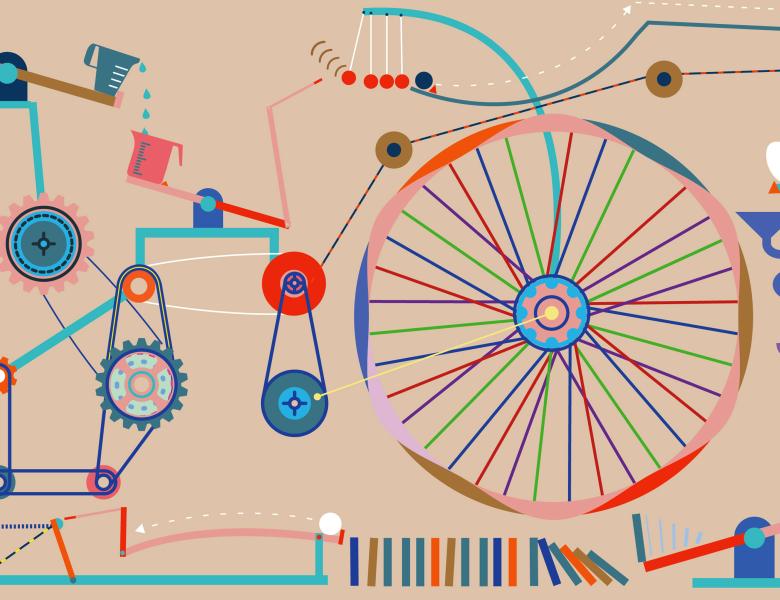
Title: Causality-inspired ML: what can causality do for ML? The domain adaptation case
Abstract: Applying machine learning to real-world cases often requires methods that are robust w.r.t. heterogeneity, missing not at random or corrupt data, selection bias, non i.i.d. data etc. and that can generalize across different domains. Moreover, many tasks are inherently trying to answer causal questions and gather actionable insights, a task for which correlations are usually not enough. Several of these issues are addressed in the rich causal inference literature. On the other hand, often classical causal inference methods require either a complete knowledge of a causal graph or enough experimental data (interventions) to estimate it accurately.
Recently, a new line of research has focused on causality-inspired machine learning, i.e. on the application ideas from causal inference to machine learning methods without necessarily knowing or even trying to estimate the complete causal graph. In this talk, I will present an example of this line of research in the unsupervised domain adaptation case, in which we have labelled data in a set of source domains and unlabelled data in a target domain ("zero-shot"), for which we want to predict the labels. In particular, given certain assumptions, our approach is able to select a set of provably "stable" features (a separating set), for which the generalization error can be bound, even in case of arbitrarily large distribution shifts. As opposed to other works, it also exploits the information in the unlabelled target data, allowing for some unseen shifts w.r.t. to the source domains. While using ideas from causal inference, our method never aims at reconstructing the causal graph or even the Markov equivalence class, showing that causal inference ideas can help machine learning even in this more relaxed setting.
All scheduled dates:
Upcoming
No Upcoming activities yet