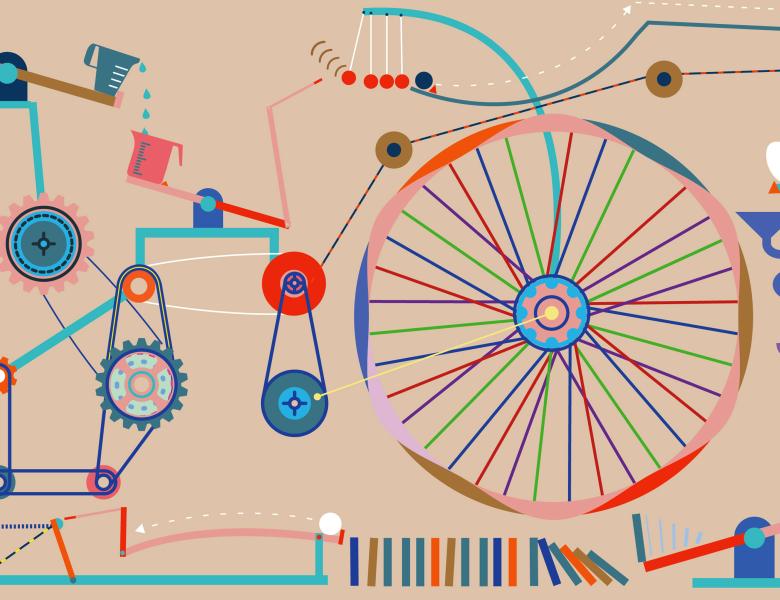
ABSTRACT:
A probability distribution over the Boolean cube is monotone if flipping the value of a coordinate from zero to one can only increase the probability of an element. Given samples of an unknown monotone distribution over the Boolean cube, we give an algorithm that learns an approximation of the distribution in statistical distance. If n is the dimension, the naive approach requires \Omega(2^n) samples, while our algorithm only needs O(2^n/2^{n^{1/5}}) samples.
To do this, we develop a structural lemma describing monotone probability distributions. The structural lemma has further implications to the sample complexity of basic testing tasks for analyzing monotone probability distributions over the Boolean cube: We use it to give nontrivial upper bounds on the tasks of estimating the distance of a monotone distribution to uniform and of estimating the support size of a monotone distribution. In the setting of monotone probability distributions over the Boolean cube, our algorithms are the first to have sample complexity lower than known lower bounds for the same testing tasks on arbitrary (not necessarily monotone) probability distributions.
One further consequence of our learning algorithm is an improved sample complexity for the task of testing whether a distribution on the Boolean cube is monotone.
(Joint work with Ronitt Rubinfeld)
BIOGRAPHY:
Arsen Vasilyan is a graduate student in computer science at MIT. His research interests include computational learning theory, distribution learning and testing, computational statistics, and algorithms more generally.
All scheduled dates:
Upcoming
No Upcoming activities yet