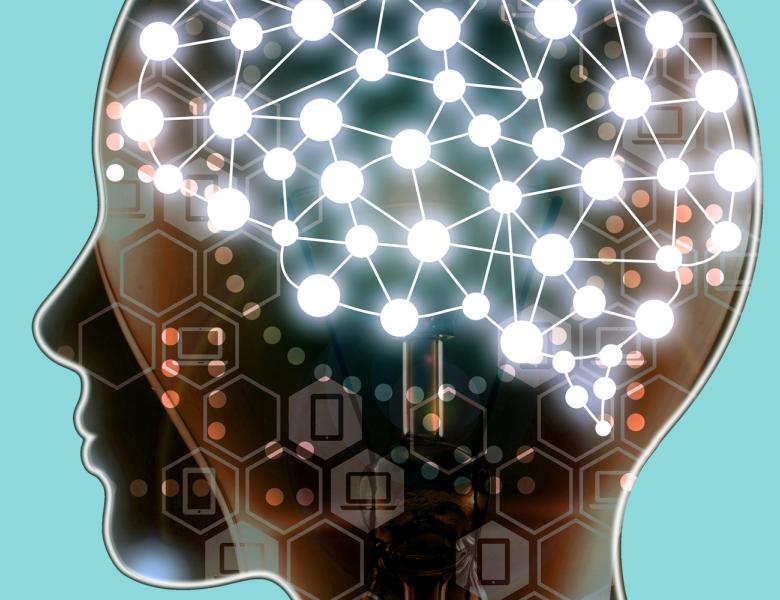
THIS EVENT HAS BEEN CANCELLED.
Abstract: Potential functions are a standard tool in the design and analysis of online learning algorithms. However, the best choice of potential function remained unclear. This talk starts with early work [Cesa-Bianchi et al 1996] that identified a min-max optimal potential function for the problems of online {0,1} predictions where the best expert is known to make at most $k$ mistakes. We extend the analysis to DTOL and derive a backwards recursion for time-dependent potentials. We extend the game to continuous time and show that for any potential function with strictly positive four derivatives the min/max optimal adversarial strategy is Brownian motion. The min/max optimal learner strategy is the best response to Brownian motion. Using this analysis the Normal-Hedge potential [Chaudhuri et al 2009, Luo, Schapire 2015] provides an almost optimal bound for unknown horizon, and we derive second order bounds for easy sequences.
Please note: the Simons Institute regularly captures photos and video of activity around the Institute for use in videos, publications, and promotional materials.
If you require special accommodation, please contact our access coordinator at simonsevents@berkeley.edu with as much advance notice as possible.
All scheduled dates:
Upcoming
No Upcoming activities yet