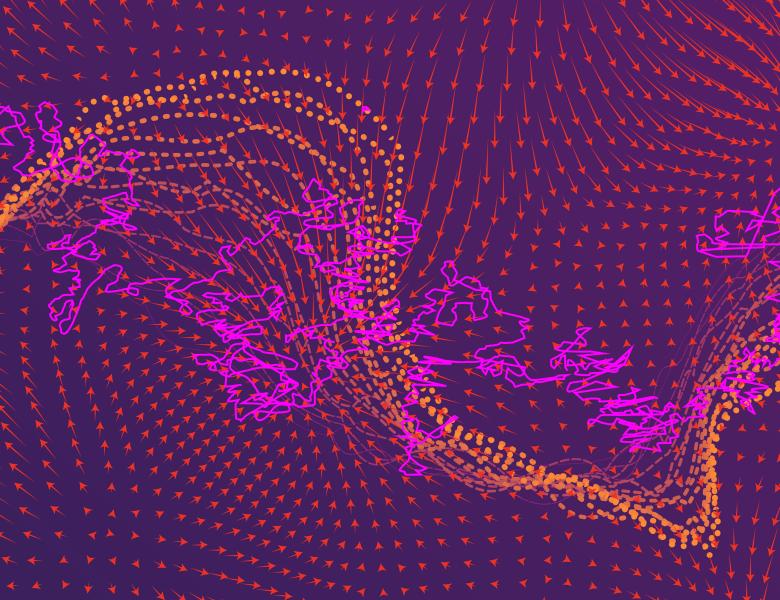
The seminal paper of Jordan, Kinderlehrer, and Otto has profoundly reshaped our understanding of sampling algorithms. What is now commonly known as the JKO scheme interprets the evolution of marginal distributions of a Langevin diffusion as a gradient flow of a Kullback-Leibler (KL) divergence over the Wasserstein space of probability measures. This optimization perspective on Markov chain Monte Carlo (MCMC) has not only renewed our understanding of algorithms based on Langevin diffusions, but has also fueled the discovery of new MCMC algorithms inspired by the diverse and powerful optimization toolbox.
The goal of this workshop is to bring together researchers from various fields (theoretical computer science, optimization, probability, statistics, and calculus of variations) to interact around new ideas that exploit this powerful framework.
This event will be held in person and virtually
Krishnakumar Balasubramanian (UC Davis), Peter Bartlett (UC Berkeley), Yuansi Chen (Duke University), Zongchen Chen (Georgia Institute of Technology), Kathleen Craig (UC Santa Barbara), Arnak Dalalyan (ENSAE Paris), Simone Di Marino (Università di Genova and Massachusetts Institute of Technology), Jelena Diakonikolas (University of Wisconsin-Madison), Paromita Dubey (Stanford), Alain Durmus (ENS Paris-Saclay), Murat Erdogdu (University of Toronto), Ronan Fleming (Leiden University), William Franks (MIT), Wilfrid Gangbo (UCLA), Nicolas Garcia Trillos (University of Wisconsin Madison), Augusto Gerolin (Vrije Universiteit Amsterdam), Quanquan Gu (University of California, Los Angeles), Franca Hoffmann (University of Bonn), Susan Holmes (Stanford), Ravindran Kannan (Microsoft Research India), Anna Korba (ENSAE Paris), Aditi Laddha (Georgia Institute of Technology), Thibaut Le Gouic (Ecole Centrale), Qi Lei (Princeton University), Qin Li (UW Madison), Jianfeng Lu (Duke University), Giulia Luise (University College London), Yian Ma (UCSD), Oren Mangoubi (WPI), Jonathan Mattingly (Duke University), Andrea Montanari (Stanford University), Eric Moulines (Ecole Polytechnique), Hari Narayanan (Tata Institute, Mumbai), Vidyut Naware (PayPal), Robin Neumayer (Northwestern University), Felix Otto (Max Planck Institute for Mathematics in the Sciences), Vianney Perchet (Université Paris Diderot - Paris 7), Natesh Pillai (Harvard), Philippe Rigollet (MIT), Andrej Risteski (Carnegie Mellon University), Ruoqi Shen (University of Washington, Seattle), Dejan Slepcev (Carnegie Mellon University), Sui Tang (UCSB), Prasad Tetali (Georgia Institute of Technology), Matthew Thorpe (University of Manchester), Kevin Tian (Stanford University), Belinda Tzen (UIUC), Santosh Vempala (Georgia Institute of Technology), Nisheeth Vishnoi (Yale University), Jiangping Wang (PayPal), Andre Wibisono (Yale University), Stephen Wright (University of Wisconsin-Madison)