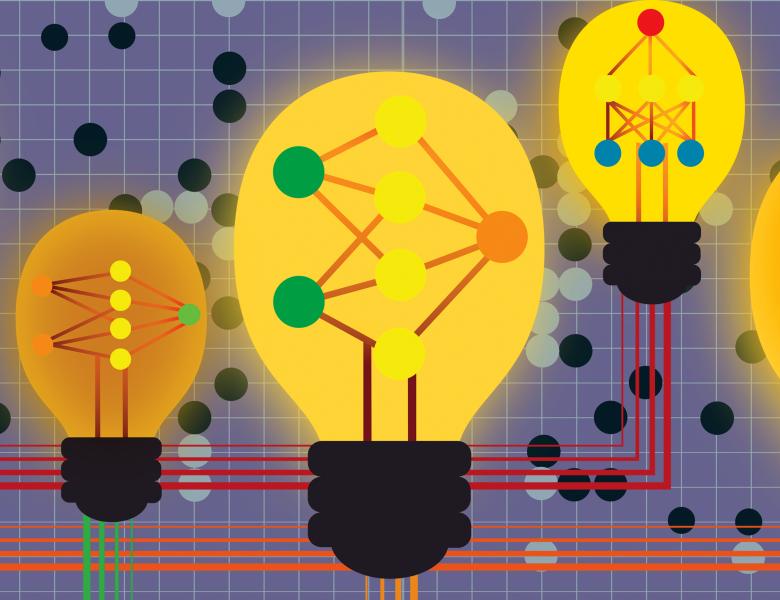
Many recent machine learning approaches have moved from an optimization perspective to an "equilibration" perspective, where a good model is framed as the equilibrium of a game, as opposed to the minimizer of an objective function. Examples include generative adversarial networks, adversarial robustness, and fairness in machine learning. While recent years have seen great progress in nonconvex optimization, with celebrated methods such stochastic gradient descent, Adagrad, and Adam driving much of the progress in deep learning, our ability to solve min-max optimization problems and finding equilibria in smooth games remains rather poor (especially when payoffs are nonconvex/concave or noisy). This workshop will bring together practitioners from these fields to discuss the practical challenges, as well as researchers working on the foundations of stochastic optimization and algorithmic game theory.