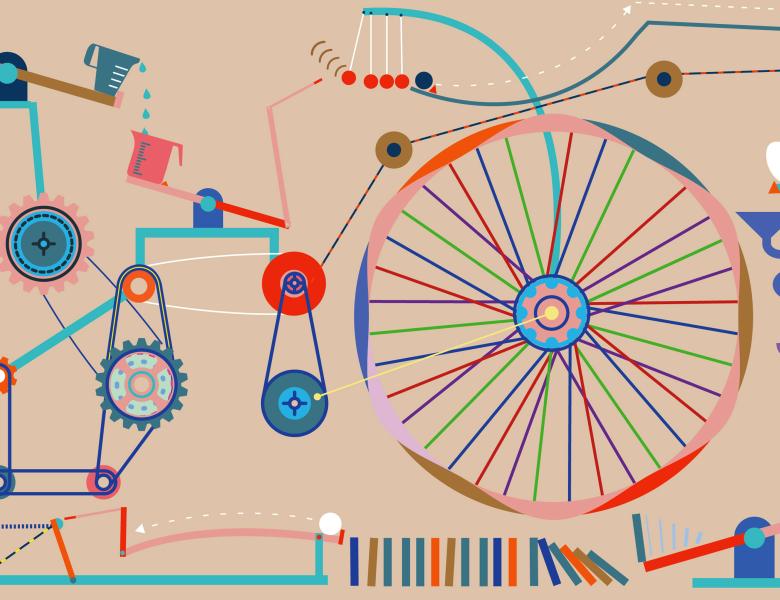
Robustness is a desideratum of any inference technique, but there are several issues specific to causality that we believe would benefit from an interaction between researchers on causality and in theoretical computer science. Among them are:
- unobserved confounding
- inter-unit causation (or "interference")
- relational or logical constraints among the variables
- heterogeneous treatment effects
- sample selection bias
- missing data (not at random)
- interventions with off-target effects
- non-stationarity and dynamical systems
Combined with the trade-off between statistical reliability and computational complexity, these challenges pose formidable hurdles to the development of robust causal inference methods.
This workshop aims to build on the quite-well-established theoretical and "in principle" understanding of these challenges by integrating various techniques from theoretical computer science to approximate optimal results and quantify uncertainty.
Anish Agarwal (MIT), Bryon Aragam (University of Chicago), Eitan Bachmat (Ben-Gurion University), Ran Balicer (Clalit Health Services), Noam Barda (Harvard Medical School), Arnab Bhattacharyya (National University of Singapore, Singapore), Emma Brunskill (Stanford University), Patrick Burauel (Caltech), Silvia Chiappa (DeepMind), Benedicte Colnet (Inria), James Cussens (University of Bristol), Yuval Dagan (MIT), Noa Dagan (Harvard Medical School), Xiaowu Dai (UC Berkeley), Constantinos Daskalakis (Massachusetts Institute of Technology), Ilias Diakonikolas (University of Wisconsin-Madison), Vanessa Didelez (Leibniz Institute, University of Bremen), Sanghamitra Dutta (JP Morgan), Frederick Eberhardt (Caltech), Robin Evans (University of Oxford), Maria Glymour (UCSF), Themistoklis Gouleakis (MIT), Fangjian Guo (University of Cambridge), Moritz Hardt (UC Berkeley), Christopher Harshaw (Yale University), Christina Heinze-Deml (ETH Zurich), Benjamin Jantzen (Virginia Tech), Julie Josse (Polytechnique), Nathan Kallus (Cornell University), Anthimos Vardis Kandiros (MIT), Shiva Kasiviswanathan (Amazon), Nur Kaynar (UCLA), Batya Kenig (Technion), Christoph Kern (University of Mannheim), Frederic Koehler (Massachusetts Institute of Technology), Issa Kohler-Hausmann (Yale Law School), DIMITRIOS MYRISIOTIS (National University of Singapore), Marloes Maathuis (Seminar for Statistics, ETH Zurich), Daniel Malinsky (Columbia University), Raghu Meka (UC Los Angeles), Karthika Mohan (Oregon State University), Ankur Moitra (Massachusetts Institute of Technology), Ismael Mourifie (University of Toronto), Betsy Ogburn (John Hopkins), Yuval Rabani (The Hebrew University of Jerusalem), Aditi Raghunathan (Stanford), Kavita Ramanan (Brown University), Omer Reingold (Stanford University), Thomas Richardson (University of Washington), Sherri Rose (Stanford University), Guy Rothblum (Weizmann Institute), Dominik Rothenhaeusler (Stanford), Andrea Rotnitzky (Torcuato Di Tella University), Leonard Schulman (Caltech), Bernhard Schölkopf (Max Planck Institute for Intelligent Systems), Devavrat Shah (Massachusetts Institute of Technology), Abhishek Shetty (UC Berkeley), Kirankumar Shiragur (Stanford University), Ilya Shpitser (Johns Hopkins University), Rahul Singh (MIT), Piyush Srivastava (Tata Institute of Fundamental Research), Vasilis Syrgkanis (Microsoft Research), Johannes Textor (Radboud University Nijmegen, The Netherlands), Jin Tian (Iowa State University), Guy Van den Broeck (UCLA), Stefan Wager (Stanford Graduate School of Business), Yuhao Wang (National University of Singapore (NUS)), Gal Yona (Weizmann Institute), Christina Yu (Cornell University), Angela Zhou (Cornell University)