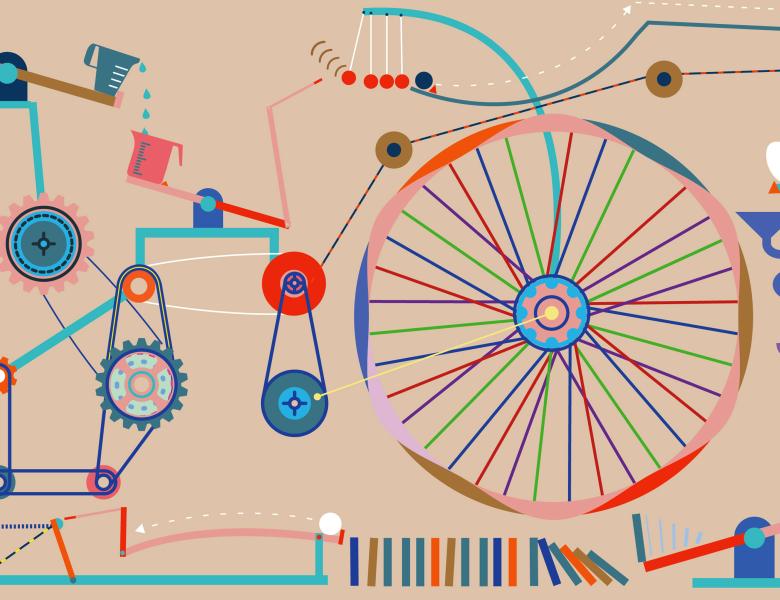
During the last decade, we have witnessed a paradigm shift in the data that may be collected. While performing large-scale randomized experiments has long been prohibitively expensive, the possibility of large-scale experiments — for example, using CRISPR perturbations in the biomedical sciences, field experiments in the social sciences, and digital experimentation — has led to a complete change in the type of data that can be obtained at scale. Observational data, even accompanied by strong assumptions, can paint only a partial picture of the underlying causal mechanisms. However, with sufficient interventional data, causal effects can be learned with much weaker assumptions.
With the floodgates open, the challenge is to control the deluge: the main difficulty in these applications lies in the heterogeneous treatment effects in large-scale experiments as well as the combinatorial explosion of the possible interventions that can be performed including experimental design in dynamical systems, sequential designs, and online experimentation. Despite the vast increase in possibilities, it remains, in general, simply not feasible to perform all possible interventions. It is therefore critical to develop a theory of experimental design for causal inference that informs which experiments to run in order to extract as much causal information as possible.
This workshop aims to integrate ideas from experimental design, active learning, policy learning, and contextual bandits, and showcase empirical work of interventions for causal inference in the social sciences, biology, medicine, and beyond.
Anish Agarwal (MIT), Kartik Ahuja (University of Montreal), P. M. Aronow (Yale University), Elias Bareinboim (Columbia University), Arnab Bhattacharyya (National University of Singapore, Singapore), Patrick Burauel (Caltech), XianJun, Davin Choo (National University of Singapore (NUS)), James Cussens (University of Bristol), Yuval Dagan (MIT), Xiaowu Dai (UC Berkeley), Vanessa Didelez (Leibniz Institute, University of Bremen), Peng Ding (University of California, Berkeley), Sandrine Dudoit (UC Berkeley), Sanghamitra Dutta (JP Morgan), Raaz Dwivedi (Harvard University), Frederick Eberhardt (Caltech), Robin Evans (University of Oxford), Avi Feller (UC Berkeley), Emily Fox (Stanford), Alison Gopnik (UC Berkeley), Fangjian Guo (University of Cambridge), Christopher Harshaw (Yale University), Kuang-Yu Hsu (University of Victoria), Nathan Kallus (Cornell University), Anthimos Vardis Kandiros (MIT), Hyunseung Kang (University of Wisconsin Madison), Shiva Kasiviswanathan (Amazon), Nur Kaynar (UCLA), Murat Kocaoglu (Purdue Univeristy), Ron Kohavi (), Issa Kohler-Hausmann (Yale Law School), Jennifer Listgarten (UC Berkeley), Marloes Maathuis (Seminar for Statistics, ETH Zurich), Luke Miratrix (Harvard), Cecilia Hyunjung Mo (UC Berkeley), Karthika Mohan (Oregon State University), Ismael Mourifie (University of Toronto), Razieh Nabi (Emory University), Molly Offer-Westort (University of Chicago), Betsy Ogburn (John Hopkins), Georgia Papadogeorgou (University of Florida), Emilija Perković (University of Washington), Maya Petersen (UC Berkeley), Kavita Ramanan (Brown University), Thomas Richardson (University of Washington), James Robins (Harvard University), Dominik Rothenhaeusler (Stanford), Andrea Rotnitzky (Torcuato Di Tella University), Chiara Sabatti (Stanford University), Jas Sekhon (Yale University), Devavrat Shah (Massachusetts Institute of Technology), Uri Shalit (Technion - Israel Institute of Technology), Karthikeyan Shanmugam (IBM), Dennis Shen (UC Berkeley), Kirankumar Shiragur (Stanford University), Rahul Singh (MIT), Liam Solus (KTH), Peter Spirtes (Carnegie Mellon University), Chandler Squires (), Scott Sussex (ETH Zurich), Vasilis Syrgkanis (Microsoft Research), Fredrik Sävje (Yale University), Yan Shuo Tan (UC Berkeley), Johannes Textor (Radboud University Nijmegen, The Netherlands), Jin Tian (Iowa State University), Caroline Uhler (MIT), Jingshen Wang (UC Berkeley), Ting Ye (University of Washington), Bin Yu (UC Berkeley), Kun Zhang (Carnegie Mellon University), Angela Zhou (Cornell University), Mirthe van Diepen (Radboud University)