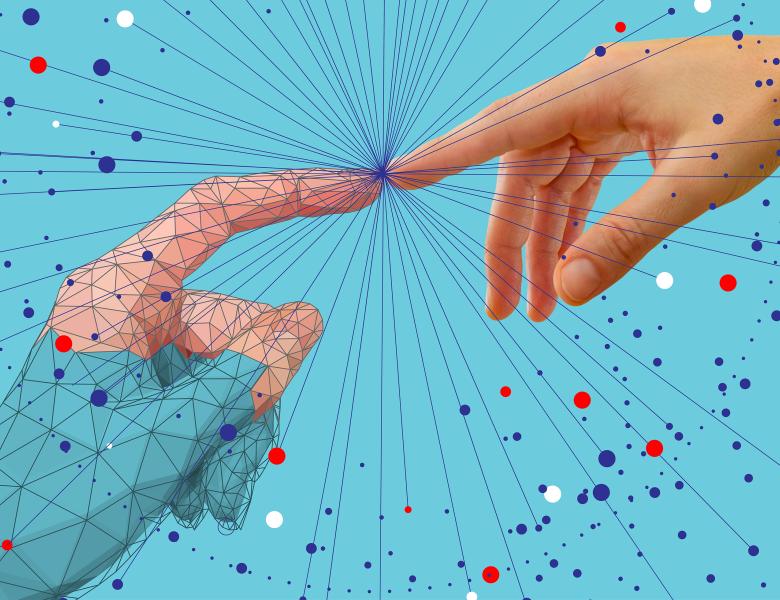
Playlist: 21 videos
AI and Humanity
0:16:50
Peter Hershock (East-West Center)
https://simons.berkeley.edu/talks/intelligent-technology-and-attention-economy-buddhist-perspective-risks-consciousness-hacking
AI and Humanity
The informational synthesis of purpose-generating, carbon-based human intelligence with purpose-implementing, silicon-based computational intelligence is widely heralded as driving a 4th Industrial Revolution, but this revolution is as ontological as it is industrial, and as ethical as it is technical. This talk makes a case for seeing: 1] that AI ethics is being hampered by default presuppositions about ontologically individual moral agents, actions, and patients; 2] that taking the human risks and rewards of intelligent technology fully into account requires a critical distinction between tools and technologies; and 3] that the greatest threat intelligent technology poses to humanity is not a technological singularity, but an ethical singularity: a collapse of the opportunity space for practicing the evaluative art of human course correction as an ironic consequence of choice-mediated attention exploitation and consciousness hacking.
Visit talk page
https://simons.berkeley.edu/talks/intelligent-technology-and-attention-economy-buddhist-perspective-risks-consciousness-hacking
AI and Humanity
The informational synthesis of purpose-generating, carbon-based human intelligence with purpose-implementing, silicon-based computational intelligence is widely heralded as driving a 4th Industrial Revolution, but this revolution is as ontological as it is industrial, and as ethical as it is technical. This talk makes a case for seeing: 1] that AI ethics is being hampered by default presuppositions about ontologically individual moral agents, actions, and patients; 2] that taking the human risks and rewards of intelligent technology fully into account requires a critical distinction between tools and technologies; and 3] that the greatest threat intelligent technology poses to humanity is not a technological singularity, but an ethical singularity: a collapse of the opportunity space for practicing the evaluative art of human course correction as an ironic consequence of choice-mediated attention exploitation and consciousness hacking.
0:47:0
Smitha Milli (UC Berkeley)
https://simons.berkeley.edu/talks/recommender-system-alignment
AI and Humanity
Most recommendation engines today are based on predicting user engagement, e.g. predicting whether a user will click on an item or not. However, there is potentially a large gap between engagement signals and a desired notion of "value" that is worth optimizing for. We use the framework of measurement theory to (a) confront the designer with a normative question about what the designer values, (b) provide a general latent variable model approach that can be used to operationalize the target construct and directly optimize for it, and (c) guide the designer in evaluating and revising their operationalization. We implement our approach on the Twitter platform on millions of users. In line with established approaches to assessing the validity of measurements, we perform a qualitative evaluation of how well our model captures a desired notion of "value".
Visit talk page
https://simons.berkeley.edu/talks/recommender-system-alignment
AI and Humanity
Most recommendation engines today are based on predicting user engagement, e.g. predicting whether a user will click on an item or not. However, there is potentially a large gap between engagement signals and a desired notion of "value" that is worth optimizing for. We use the framework of measurement theory to (a) confront the designer with a normative question about what the designer values, (b) provide a general latent variable model approach that can be used to operationalize the target construct and directly optimize for it, and (c) guide the designer in evaluating and revising their operationalization. We implement our approach on the Twitter platform on millions of users. In line with established approaches to assessing the validity of measurements, we perform a qualitative evaluation of how well our model captures a desired notion of "value".
0:16:45
Kacper Sokol (RMIT University)
https://simons.berkeley.edu/talks/tbd-453
AI and Humanity
A myriad of approaches exists to help us peer inside automated decision-making systems based on artificial intelligence and machine learning algorithms. These tools and their insights, however, are socio-technological constructs themselves, hence subject to human biases and preferences as well as technical limitations. Under these conditions, how can we ensure that explanations are meaningful and fulfil their role by leading to understanding? In this talk I will demonstrate how different configurations of an explainability algorithm may impact the resulting insights and show the importance of the strategy employed to present them to the user, arguing in favour of a clear separation between the technical and social aspects of such tools.
Visit talk page
https://simons.berkeley.edu/talks/tbd-453
AI and Humanity
A myriad of approaches exists to help us peer inside automated decision-making systems based on artificial intelligence and machine learning algorithms. These tools and their insights, however, are socio-technological constructs themselves, hence subject to human biases and preferences as well as technical limitations. Under these conditions, how can we ensure that explanations are meaningful and fulfil their role by leading to understanding? In this talk I will demonstrate how different configurations of an explainability algorithm may impact the resulting insights and show the importance of the strategy employed to present them to the user, arguing in favour of a clear separation between the technical and social aspects of such tools.
0:42:40
Megha Srivastava (Stanford University)
https://simons.berkeley.edu/talks/assistive-teaching-motor-control-tasks-humans
AI and Humanity
Recent works on shared autonomy and assistive-AI technologies, such as assistive robotic teleoperation, seek to model and help human users with limited ability in a fixed task. However, these approaches often fail to account for humans' ability to adapt and eventually learn how to execute a control task themselves. Furthermore, in applications where it may be desirable for a human to intervene, these methods may have inhibited their ability to learn how to succeed with full self-control.We focus on the problem of assistive teaching of motor control tasks such as parking a car or landing an aircraft. Despite their ubiquitous role in humans' daily activities and occupations, motor tasks are rarely taught in a uniform way due to their high complexity and variance. We propose an AI-assisted teaching algorithm that leverages skill discovery methods from reinforcement learning (RL) literature to (i) break down any motor control task into teachable skills, (ii) construct novel drill sequences, and (iii) individualize curricula to students with different capabilities. We show that AI-assisted teaching with skills improve student performance by around 40% compared to practicing full trajectories without assistance, and practicing with individualized drills can result in up to 25% further improvement.
Visit talk page
https://simons.berkeley.edu/talks/assistive-teaching-motor-control-tasks-humans
AI and Humanity
Recent works on shared autonomy and assistive-AI technologies, such as assistive robotic teleoperation, seek to model and help human users with limited ability in a fixed task. However, these approaches often fail to account for humans' ability to adapt and eventually learn how to execute a control task themselves. Furthermore, in applications where it may be desirable for a human to intervene, these methods may have inhibited their ability to learn how to succeed with full self-control.We focus on the problem of assistive teaching of motor control tasks such as parking a car or landing an aircraft. Despite their ubiquitous role in humans' daily activities and occupations, motor tasks are rarely taught in a uniform way due to their high complexity and variance. We propose an AI-assisted teaching algorithm that leverages skill discovery methods from reinforcement learning (RL) literature to (i) break down any motor control task into teachable skills, (ii) construct novel drill sequences, and (iii) individualize curricula to students with different capabilities. We show that AI-assisted teaching with skills improve student performance by around 40% compared to practicing full trajectories without assistance, and practicing with individualized drills can result in up to 25% further improvement.
0:16:50
Ben Green (University of Michigan)
https://simons.berkeley.edu/talks/flaws-policies-requiring-human-oversight-government-algorithms
AI and Humanity
As algorithms become an influential component of government decision-making around the world, policymakers have debated how governments can attain the benefits of algorithms while preventing the harms of algorithms. One mechanism that has become a centerpiece of global efforts to regulate government algorithms is to require human oversight of algorithmic decisions. Despite the widespread turn to human oversight, these policies rest on an uninterrogated assumption: that people are able to effectively oversee algorithmic decision-making. In this article, I survey 41 policies that prescribe human oversight of government algorithms and find that they suffer from two significant flaws. First, evidence suggests that people are unable to perform the desired oversight functions. Second, as a result of the first flaw, human oversight policies legitimize government uses of faulty and controversial algorithms without addressing the fundamental issues with these tools. Thus, rather than protect against the potential harms of algorithmic decision-making in government, human oversight policies provide a false sense of security in adopting algorithms and enable vendors and agencies to shirk accountability for algorithmic harms. In light of these flaws, I propose a shift from human oversight to institutional oversight as the central mechanism for regulating government algorithms. This institutional approach operates in two stages. First, agencies must justify that it is appropriate to incorporate an algorithm into decision-making and that any proposed forms of human oversight are supported by empirical evidence. Second, these justifications must receive democratic public review and approval before the agency can adopt the algorithm.
Visit talk page
https://simons.berkeley.edu/talks/flaws-policies-requiring-human-oversight-government-algorithms
AI and Humanity
As algorithms become an influential component of government decision-making around the world, policymakers have debated how governments can attain the benefits of algorithms while preventing the harms of algorithms. One mechanism that has become a centerpiece of global efforts to regulate government algorithms is to require human oversight of algorithmic decisions. Despite the widespread turn to human oversight, these policies rest on an uninterrogated assumption: that people are able to effectively oversee algorithmic decision-making. In this article, I survey 41 policies that prescribe human oversight of government algorithms and find that they suffer from two significant flaws. First, evidence suggests that people are unable to perform the desired oversight functions. Second, as a result of the first flaw, human oversight policies legitimize government uses of faulty and controversial algorithms without addressing the fundamental issues with these tools. Thus, rather than protect against the potential harms of algorithmic decision-making in government, human oversight policies provide a false sense of security in adopting algorithms and enable vendors and agencies to shirk accountability for algorithmic harms. In light of these flaws, I propose a shift from human oversight to institutional oversight as the central mechanism for regulating government algorithms. This institutional approach operates in two stages. First, agencies must justify that it is appropriate to incorporate an algorithm into decision-making and that any proposed forms of human oversight are supported by empirical evidence. Second, these justifications must receive democratic public review and approval before the agency can adopt the algorithm.
0:48:0
Connal Parsley (University of Kent)
https://simons.berkeley.edu/talks/tbd-451
AI and Humanity
In this talk, I introduce the project ‘The Future of Good Decisions: An evolutionary approach to human-AI government administrative decision-making’, recently funded by a UK fellowship scheme to run from 2022-2029. This project addresses the impasse between automated decision-making and core values of the rule of law (like fairness and transparency). Moving past today’s dominant question of whether machine learning technologies can be made to conform to legal criteria, or a new regulatory paradigm should be defined by data science, it asks: how can our ideas of good administrative decisions evolve for the coming age when humans and machines are indistinguishable? The project aims to articulate conceptions of decision quality that are appropriate to evolving technosocial ecologies; to integrate those conceptions with contemporary legal theory and jurisprudence; and to identify reform to administrative decision practices and related legal doctrines. This talk has three main aims. First, I will orient the project’s overall approach in relation to dominant strategies to protect administrative decision-making, including ‘human in the loop’. Second, I will outline its unique multi-method research design. Finally, I will explain the use of collaborative ‘Live Action Role Play’ in ‘prefiguring’ models of participatory deliberation, and in reflecting on value and quality in decision-making processes.
Visit talk page
https://simons.berkeley.edu/talks/tbd-451
AI and Humanity
In this talk, I introduce the project ‘The Future of Good Decisions: An evolutionary approach to human-AI government administrative decision-making’, recently funded by a UK fellowship scheme to run from 2022-2029. This project addresses the impasse between automated decision-making and core values of the rule of law (like fairness and transparency). Moving past today’s dominant question of whether machine learning technologies can be made to conform to legal criteria, or a new regulatory paradigm should be defined by data science, it asks: how can our ideas of good administrative decisions evolve for the coming age when humans and machines are indistinguishable? The project aims to articulate conceptions of decision quality that are appropriate to evolving technosocial ecologies; to integrate those conceptions with contemporary legal theory and jurisprudence; and to identify reform to administrative decision practices and related legal doctrines. This talk has three main aims. First, I will orient the project’s overall approach in relation to dominant strategies to protect administrative decision-making, including ‘human in the loop’. Second, I will outline its unique multi-method research design. Finally, I will explain the use of collaborative ‘Live Action Role Play’ in ‘prefiguring’ models of participatory deliberation, and in reflecting on value and quality in decision-making processes.
0:44:21
Andreea Bobu (UC Berkeley)
https://simons.berkeley.edu/talks/aligning-robot-representations-humans
AI and Humanity
As robots are increasingly deployed in real-world scenarios, a key question is how to best transfer knowledge learned in one environment to another, where shifting constraints and human preferences render adaptation challenging. A central challenge remains that often, it is difficult (perhaps even impossible) to capture the full complexity of the deployment environment, and therefore the desired tasks, at training time. Consequently, the representation, or abstraction, of the tasks the human hopes for the robot to perform in one environment may be misaligned with the representation of the tasks that the robot has learned in another. In this talk, I postulate that because humans will be the ultimate evaluator of system success in the world, they are best suited to communicating the aspects of the tasks that matter to the robot. To this end, I will discuss our insight that effective learning from human input requires first explicitly learning good intermediate representations and then using those representations for solving downstream tasks.
Visit talk page
https://simons.berkeley.edu/talks/aligning-robot-representations-humans
AI and Humanity
As robots are increasingly deployed in real-world scenarios, a key question is how to best transfer knowledge learned in one environment to another, where shifting constraints and human preferences render adaptation challenging. A central challenge remains that often, it is difficult (perhaps even impossible) to capture the full complexity of the deployment environment, and therefore the desired tasks, at training time. Consequently, the representation, or abstraction, of the tasks the human hopes for the robot to perform in one environment may be misaligned with the representation of the tasks that the robot has learned in another. In this talk, I postulate that because humans will be the ultimate evaluator of system success in the world, they are best suited to communicating the aspects of the tasks that matter to the robot. To this end, I will discuss our insight that effective learning from human input requires first explicitly learning good intermediate representations and then using those representations for solving downstream tasks.
0:17:55
Serena Wang (UC Berkeley)
https://simons.berkeley.edu/talks/tbd-454
AI and Humanity
With the rapid proliferation of machine learning technologies in the education sphere, we address an urgent need to investigate whether the development of these machine learning technologies supports holistic education principles and goals. We present findings from a cross-disciplinary interview study of education researchers, investigating whether the stated or implied "social good" objectives of ML4Ed research papers are aligned with the ML problem formulation, objectives, and interpretation of results. Our findings shed light on two main alignment gaps: the formulation of an ML problem from education goals and the translation predictions to interventions.
Visit talk page
https://simons.berkeley.edu/talks/tbd-454
AI and Humanity
With the rapid proliferation of machine learning technologies in the education sphere, we address an urgent need to investigate whether the development of these machine learning technologies supports holistic education principles and goals. We present findings from a cross-disciplinary interview study of education researchers, investigating whether the stated or implied "social good" objectives of ML4Ed research papers are aligned with the ML problem formulation, objectives, and interpretation of results. Our findings shed light on two main alignment gaps: the formulation of an ML problem from education goals and the translation predictions to interventions.
0:48:51
Yuchen Cui (Stanford)
https://simons.berkeley.edu/talks/designing-human-aware-learning-agents-understanding-relationship-between-interactions-and
AI and Humanity
Human-in-the-loop machine learning is a widely adopted paradigm for instilling human knowledge in autonomous agents. Many design choices influence the efficiency and effectiveness of such interactive learning processes, particularly the interaction type through which the human teacher may provide feedback. While different interaction types (demonstrations, preferences, etc.) have been proposed and evaluated in the HIL-ML literature, there has been little discussion of how these compare or how they should be selected to best address a particular learning problem. In this talk, I will introduce an organizing principle for interactive machine learning that provides a way to analyze the effects of interaction types on human performance and training data. I will also identify open problems in understanding the effects of interaction types.
Visit talk page
https://simons.berkeley.edu/talks/designing-human-aware-learning-agents-understanding-relationship-between-interactions-and
AI and Humanity
Human-in-the-loop machine learning is a widely adopted paradigm for instilling human knowledge in autonomous agents. Many design choices influence the efficiency and effectiveness of such interactive learning processes, particularly the interaction type through which the human teacher may provide feedback. While different interaction types (demonstrations, preferences, etc.) have been proposed and evaluated in the HIL-ML literature, there has been little discussion of how these compare or how they should be selected to best address a particular learning problem. In this talk, I will introduce an organizing principle for interactive machine learning that provides a way to analyze the effects of interaction types on human performance and training data. I will also identify open problems in understanding the effects of interaction types.
0:18:5
Thao Phan (Monash University)
https://simons.berkeley.edu/talks/race-beyond-perception-analysing-race-post-visual-regimes
AI and Humanity
In their influential introduction to racial formations, Michael Omi and Howard Winant define race as an essentially visual phenomenon. They state that “race is ocular in an irreducible way. Human bodies are visually read, understood, and narrated by means of symbolic meanings and associations. Phenotypic differences are not necessarily seen or understood in the same consistent manner across time and place, but they are nevertheless operating in specific social settings†(2015, 28). In studies of media and digital culture, moreover, processes of racialisation have most often been conceptualised as operating in visual regimes and studied using visual methods. But how do we study race when its formations are primarily figured through regimes of computation that rely on structures that are, for the most part, opaque? How do we account for processes of racialisation that operate through proxies and abstractions that figure racialized bodies not as single, coherent subjects, but as shifting clusters of data? In this paper, we discuss the challenges of researching race within algorithmic culture. We argue that previous formations of race that had been dependent on visual regimes are now giving way to structures that manage bodies through largely non-visible (or invisual) processes. In this new regime, race emerges as an epiphenomenon of processes of classifying and sorting — what we call “racial formations as data formations.†This discussion is significant because it raises new theoretical, methodological, and political questions for scholars of culture and media. This paper asks: how are we supposed to think, to identify, and to confront race and racialisation when they vanish into algorithmic systems that are beyond our perception? How do post-visual regimes disrupt the ways we have traditionally studied race? And, what methods might we use to render these processes tractable — if not “visible†— for the purposes of analysis and critique?
Visit talk page
https://simons.berkeley.edu/talks/race-beyond-perception-analysing-race-post-visual-regimes
AI and Humanity
In their influential introduction to racial formations, Michael Omi and Howard Winant define race as an essentially visual phenomenon. They state that “race is ocular in an irreducible way. Human bodies are visually read, understood, and narrated by means of symbolic meanings and associations. Phenotypic differences are not necessarily seen or understood in the same consistent manner across time and place, but they are nevertheless operating in specific social settings†(2015, 28). In studies of media and digital culture, moreover, processes of racialisation have most often been conceptualised as operating in visual regimes and studied using visual methods. But how do we study race when its formations are primarily figured through regimes of computation that rely on structures that are, for the most part, opaque? How do we account for processes of racialisation that operate through proxies and abstractions that figure racialized bodies not as single, coherent subjects, but as shifting clusters of data? In this paper, we discuss the challenges of researching race within algorithmic culture. We argue that previous formations of race that had been dependent on visual regimes are now giving way to structures that manage bodies through largely non-visible (or invisual) processes. In this new regime, race emerges as an epiphenomenon of processes of classifying and sorting — what we call “racial formations as data formations.†This discussion is significant because it raises new theoretical, methodological, and political questions for scholars of culture and media. This paper asks: how are we supposed to think, to identify, and to confront race and racialisation when they vanish into algorithmic systems that are beyond our perception? How do post-visual regimes disrupt the ways we have traditionally studied race? And, what methods might we use to render these processes tractable — if not “visible†— for the purposes of analysis and critique?