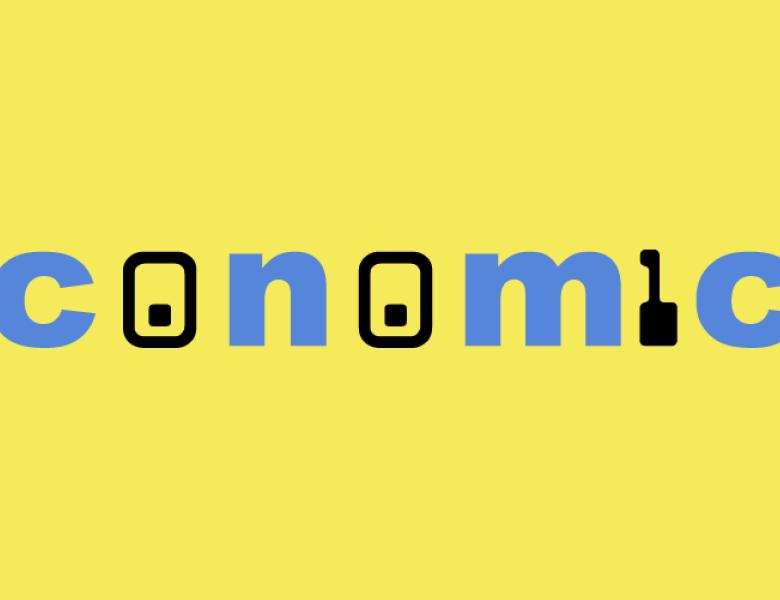
Abstract
We initiate the exploration of the use of tools from deep learning on the design of revenue optimal, dominant-strategy incentive compatible auctions. We show that multi-layer neural networks can recover optimal designs such as Myerson’s auction for a single item or Manelli and Vincent’s mechanism for a single buyer with additive preferences over two items, even with no prior knowledge about the form of optimal auctions and when the only feedback to the network are revenue and regret. We further show how known characterization results, even rather implicit ones, such as Rochet’s characterization through induced utilities and their gradients, can help speed up the learning process. We conclude by demonstrating the potential of these approaches for deriving optimal mechanisms for poorly understood problems.