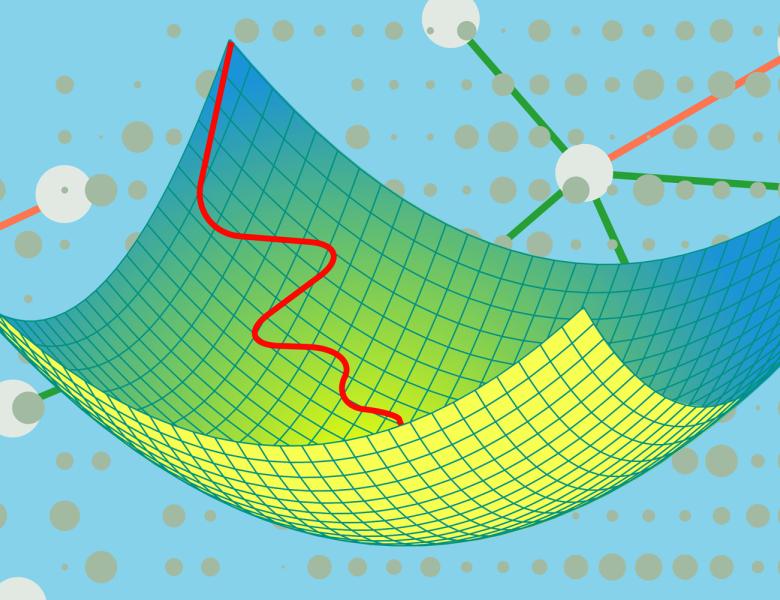
Abstract
Recently, the Linear Transformer model has received considerable attention as a proxy for understanding the behavior of the full-fledged Transformers. In particular, a number of papers have recently provided theoretical proof that Linear Transformers are able to learn the linear regression task in-context by implementing a gradient-based optimization in its forward pass. These results shed light on the mechanism through which Transformers can learn in-context in practice. In addition to covering these papers, I will also discuss some interesting empirical observations, which suggest that the optimization landscape of Linear Transformers itself may be provide a good approximation for understanding optimization of real Transformers.