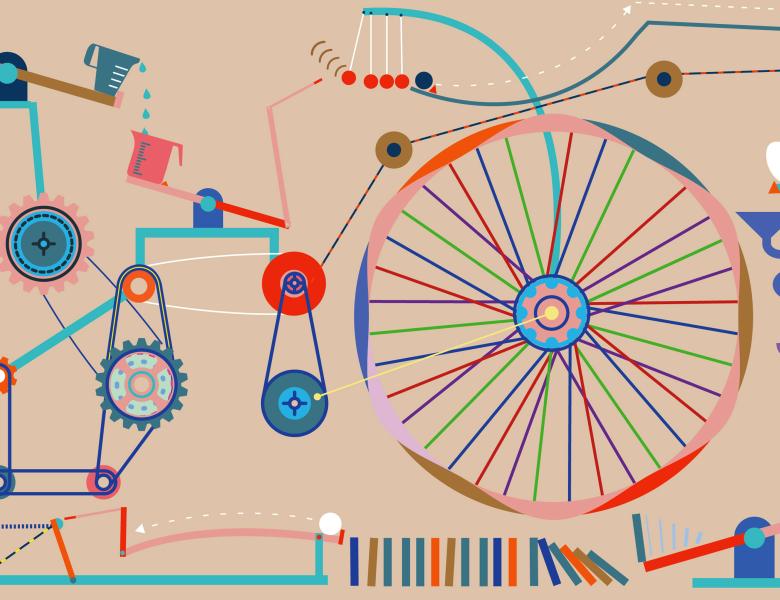
Abstract
Spatial confounding has different interpretations in the spatial and causal inference literatures. I will begin this talk by clarifying these two interpretations. Then, seeing spatial confounding through the causal inference lense, I discuss approaches to account for unmeasured variables that are spatially structured when we are interested in estimating causal effects. The first approach is based on the propensity score. In the second approach, we aim to bridge the spatial and causal inference literatures by estimating causal effects in the presence of unmeasured spatial variables using outcome modeling tools that are popular in spatial statistics.