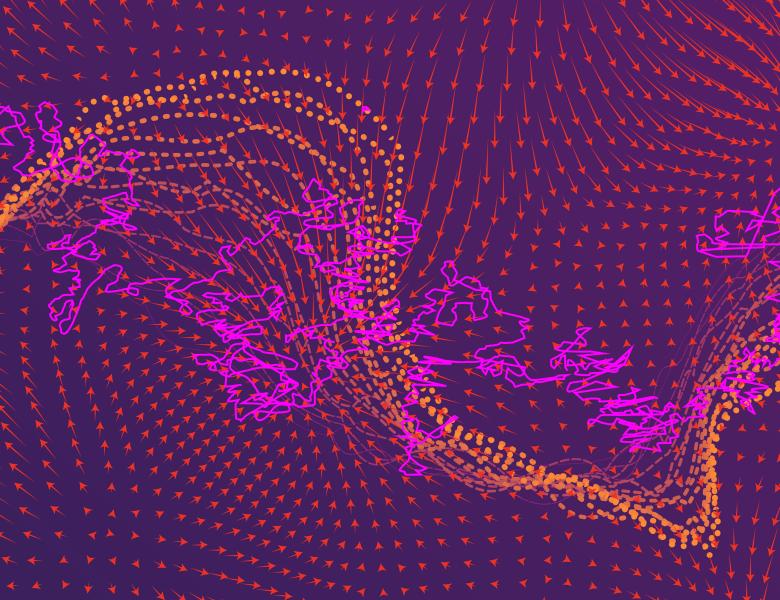
Abstract
Generative Adversarial Networks (GANs) have seen remarkable success in the field of machine learning. In the Wasserstein GAN framework, the core idea is to solve the minimax problem over the generator, and the discriminator which is restricted to be a 1-Lipschitz function. In this talk, we discuss how to enforce a 1-Lipschitz bound without the need of introducing a gradient penalty. Based on the comparison between objective functions arising in Kantorovich duality, we observe that our algorithm can compute the Wasserstein distance accurately and enforce the Lipschitz constraint well.