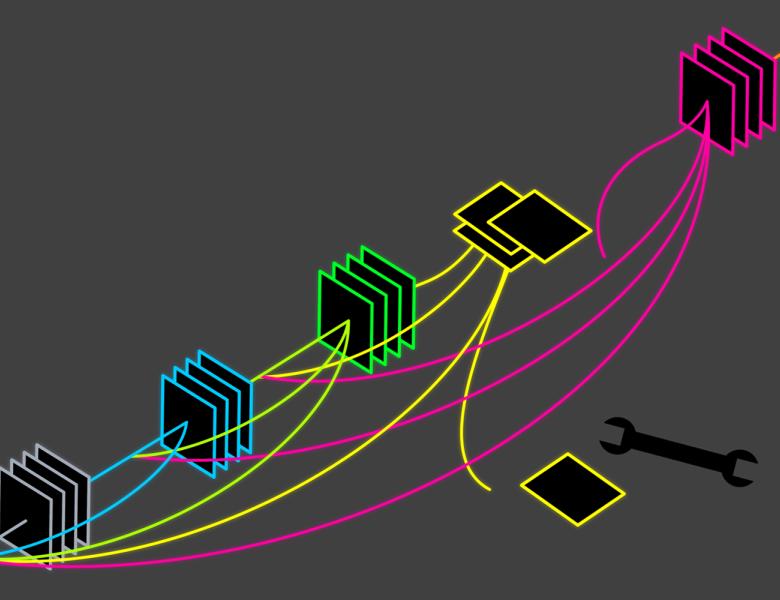
Abstract
Sample efficiency is a major challenge of applying deep reinforcement learning (RL) techniques to robotics tasks --- existing
algorithms often require a massive amount of interactions with the environment (samples). Promising solutions include model-based
reinforcement learning and imitation learning (IL). However, the theoretical understanding of them is mostly missing in the setting of
continuous and high-dimensional state space and neural network function approximators.
In this talk, I will present recent work on designing principled model-based RL and IL algorithms with theoretical analyses. These
algorithms also empirically outperform prior works on benchmark tasks in sample efficiency.
No prior knowledge of deep reinforcement learning and imitation learning is required. Based on joint works with Nick Landolfi, Yuping Luo, Garrett Thomas, Huazhe Xu, Trevor Darrell, and Yuandong Tian.