Image
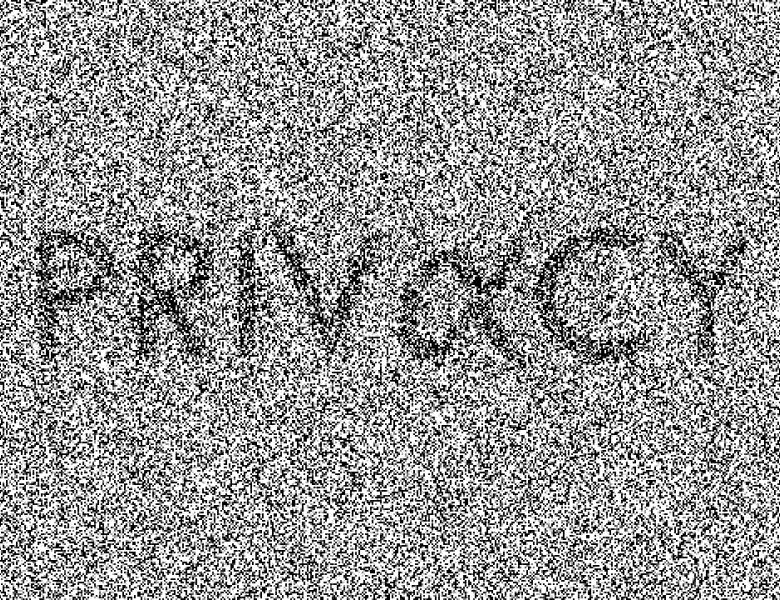
In this talk, I will present new differentially private learning algorithms that are given access to a limited amount of public unlabeled data. These algorithms use any non-private learning algorithm as a black box. We prove formal privacy and accuracy guarantees for such algorithms. The accuracy guarantees depend only on the accuracy of the underlying non-private learning algorithm. As a consequence, we prove upper bounds on the private sample complexity in the Probably Approximately Correct (PAC) learning model. These bounds are completely characterized by the VC dimension of the concept class.