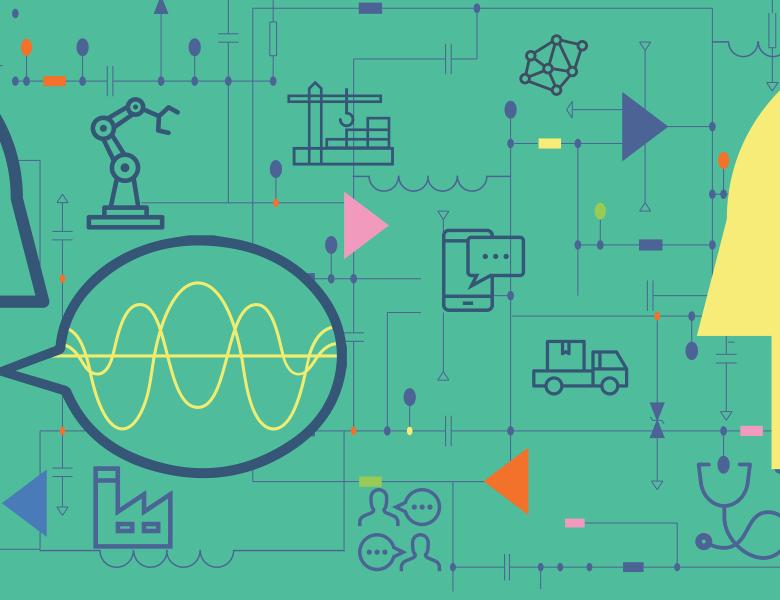
Abstract
A central problem of causal inference is the estimation of a causal effect, such as an average treatment effect, using only observational data; the do-calculus, and other tools, provide formulas that express causal effects as functionals of the observational distribution. We consider the over-identified setting where several different formulas, each utilizing different covariates, are available, and the task of the investigator is to select a formula with good large-sample performance. To assist in making this decision, the investigator may collect data and alter the data collection mechanism in a data-dependent way. We formalize this setting as a best-arm-identification bandit problem where the standard goal of learning the arm with the lowest mean is replaced with the goal of learning the arm that will produce the best estimate. We introduce new tools for constructing finite-sample confidence bounds on estimates of the asymptotic variance and show that these bounds have a favorable, second-order dependence on the rate of estimation of nuisance functions, reminiscent of the Double/Debiased Machine Learning literature. We adapt the Successive Elimination and LUCB algorithms to our setting and validate our method by providing upper bounds on the sample complexity and an empirical study on artificially generated data.