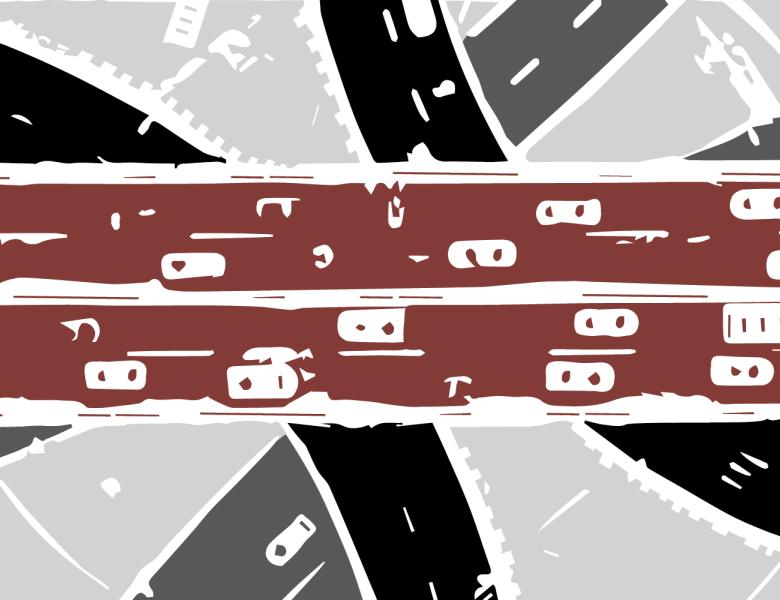
Abstract
To identify scientifically valuable objects like supernovae or asteroids on the sky, astronomical imaging surveys have historically adopted a manual approach, employing humans to visually inspect data for signatures of the events. But recent advances in the capabilities of telescopes, detectors, and supercomputers have fueled a dramatic rise in the data production rates of such surveys, straining the ability of their teams to quickly and comprehensively look at images to perform discovery. In this talk I describe how machine learning has provided a transformative solution to this astronomical problem. As an example, I describe a Random Forest approach that our group developed to automate the discovery of supernovae and other interesting objects on images, and I present results from its use in the Dark Energy Survey Supernova program (DES-SN), where it was trained using a sample of 898,963 signal and background events generated by the transient detection pipeline. After reprocessing the data collected during the first DES-SN observing season (2013 September through 2014 February) using the algorithm, the number of transient candidates eligible for human scanning decreased by a factor of 13.4, while only 1.0% of the artificial Type Ia supernovae (SNe) injected into search images to monitor survey efficiency were lost, most of which were very faint events. This advance dramatically improved the efficiency of the survey. An implementation of the algorithm and the training data are available at at http://portal.nersc.gov/project/dessn/autoscan.