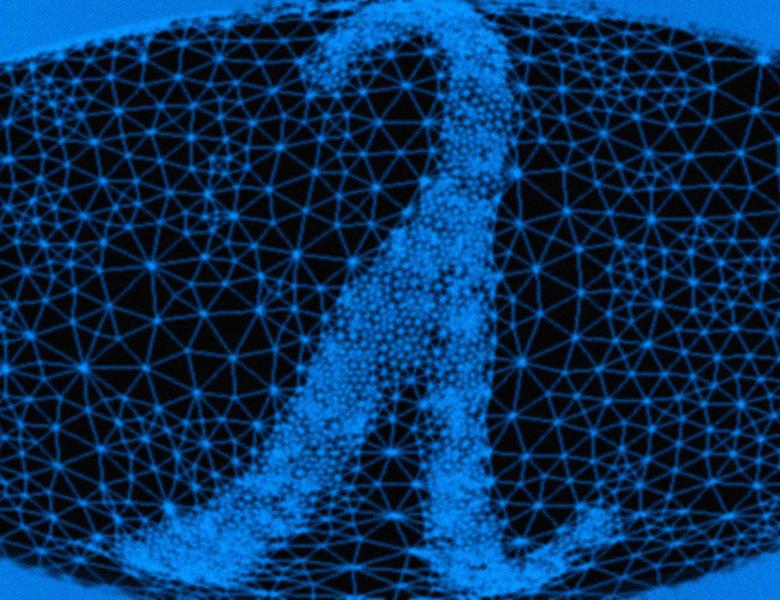
Abstract
I present on-going research on two applications where spectral methods may be helpful. The first application models human memory search as a random walk, but with a twist that only the first visit to a state is observable. The task is to estimate the transition matrix from such censored observations. The second application teaches humans a target labeling on a graph. The assumption is that, given a few initial labeled nodes in the graph, humans utilize a known algorithm to complete the labeling on the rest of the graph (think of mincut). The task is to select the smallest set of initial nodes so that the completed labeling equals the target, i.e. the inverse problem of graph-based semi-supervised learning. The goal of the talk is to generate discussions on the research challenges in these and other similar applications.