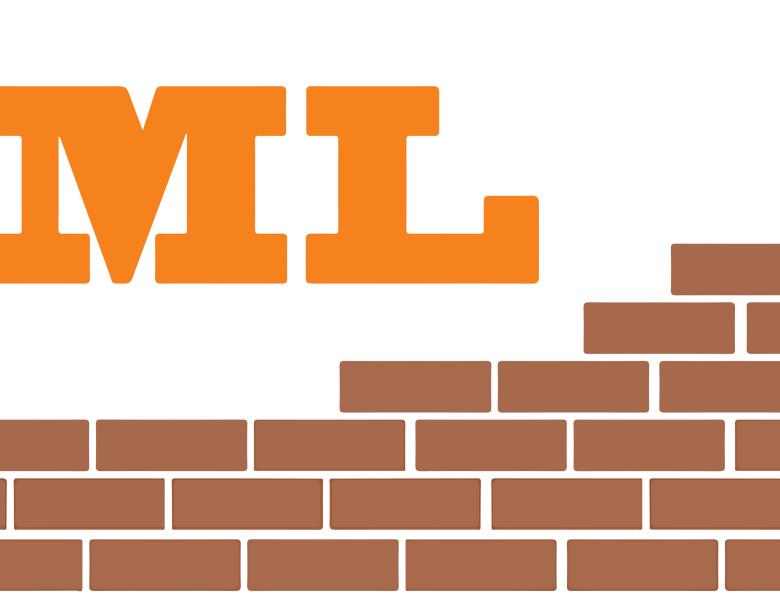
Abstract
The empirical success of Deep Learning is stunning, and everyday we hear new success stories. However, for both theoreticians and practitioners, it is important to understand the limitations. We describe three families of problems for which existing deep learning algorithms fail. We illustrate practical cases in which these failures apply and provide a theoretical insight explaining the source of difficulty.
Joint work with Ohad Shamir and Shaked Shammah.