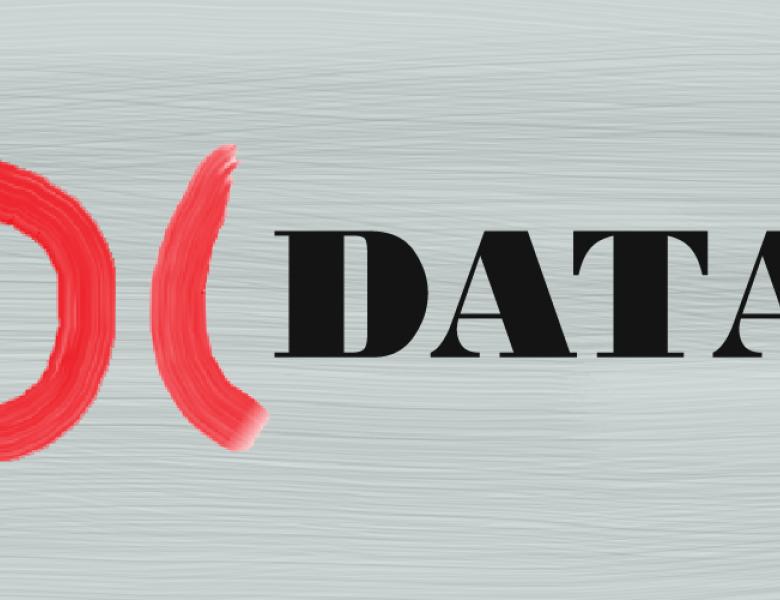
Abstract
The analysis of Big Data involves often the selection of a few promising finding out of extremely large number of potential ones. Such selection may affect our inferences about the significance, the size, and the uncertainty of the selected findings. In this tutorial I shall motivate our interest in this 'selective inference' problem, present the false discovery rate (FDR) as an effective approach to assess the significance of the selected discoveries in large scale problems, and review the available methodologies to address its control (and some open problems). I shall then carry the insight gained from FDR into selective estimation and confidence intervals, as well as model selection. I shall end by describing the currently active area of post model selection confidence intervals and estimators.