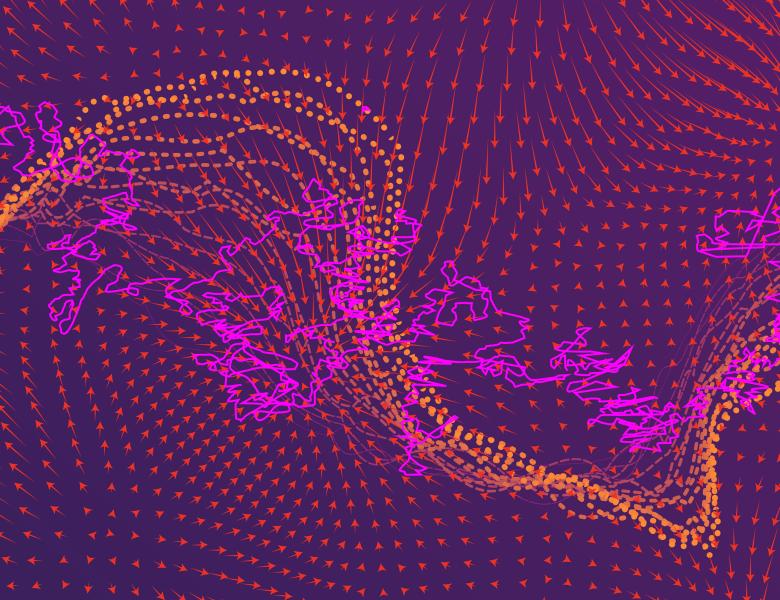
Abstract
Score-based generative modeling (SGM) is a highly successful approach for learning a probability distribution from data and generating further samples, based on learning the score function (gradient of log-pdf) and then using it to simulate a stochastic differential equation that transforms white noise into the data distribution.
We develop a convergence theory for SGM which applies to any distribution with bounded 2nd moment, relying only on a L^2-accurate score estimate, with polynomial dependence on all parameters and no reliance on smoothness or functional inequalities. We give an error of $\epsilon$ in reverse KL-divergence to the variance-$\delta$ perturbation of the data distribution with $\tilde O(d^2\log^2(1/\delta)/\epsilon^2)$ steps, and error guarantees to the exact data distribution under smoothness. Our analysis builds on the Girsanov framework by incorporating the high-probability smoothing effect of the forward diffusion and quantifies the effect of step size schedule.
Based on joint works with Hongrui Chen (Peking University), Jianfeng Lu and Yixin Tan (Duke). https://arxiv.org/abs/2211.01916