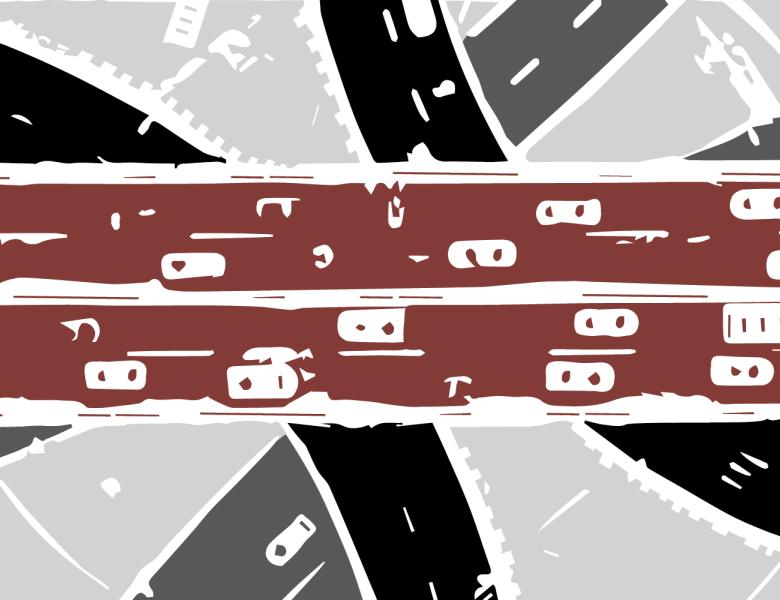
Abstract
Linear-time algorithms have long been considered the gold standard of computational efficiency. Indeed, it is hard to imagine doing better than that, since for a nontrivial problem, any algorithm must consider all of the input in order to make a decision. However, as extremely large data sets are pervasive, it is natural to wonder what information can be computed in sub-linear time. Over the past decades, several surprising advances have been made on designing such algorithms. We will give a non-exhaustive survey of this emerging area, highlighting recent progress and directions for further research. Special attention will be given to (1) sub-linear time algorithms for estimating parameters of graphs (2) local algorithms for optimization problems and (3) algorithms for estimating parameters of discrete distributions over large domains, with a number of samples that is sub-linear in the domain size.