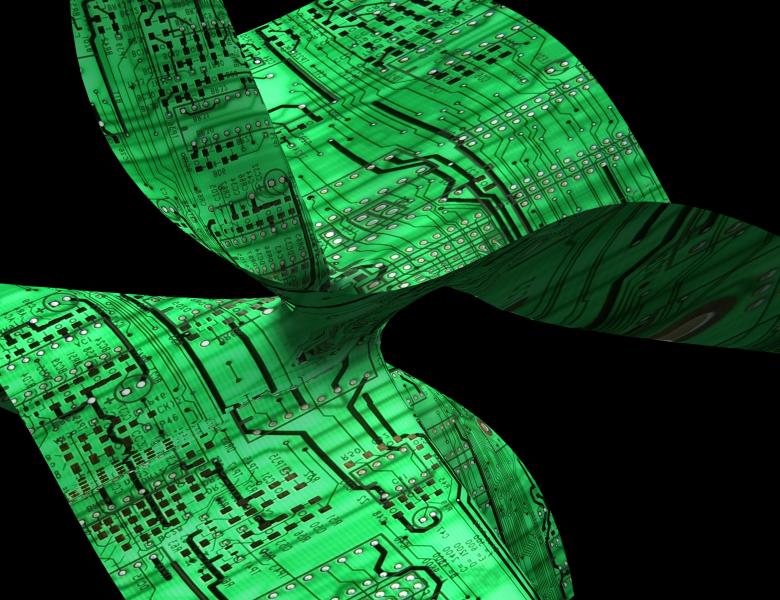
Abstract
Learning mixture of Gaussians has been a classical problem in machine learning and theoretical computer science. Many different approaches were developed, including a line of work starting from [Dasgupta 99] that works for well-separated Gaussians, and an algorithm that does not depend on separation by Moitra and Valiant. However the running time of the latter is exponential in the number of Gaussians. Recently, [Hsu Kakade 13] developed an algorithm based on method of moments that can learn k spherical Gaussians in d dimensions when k<=d, without requiring any separation.
In this talk we show it is possible to learn k Gaussians with general covariance matrices, when the dimension d is at least k^2. This involves new observations on the structure of moment tensors for general Gaussians, and new ways of doing smoothed analysis for the condition number of structured matrices.