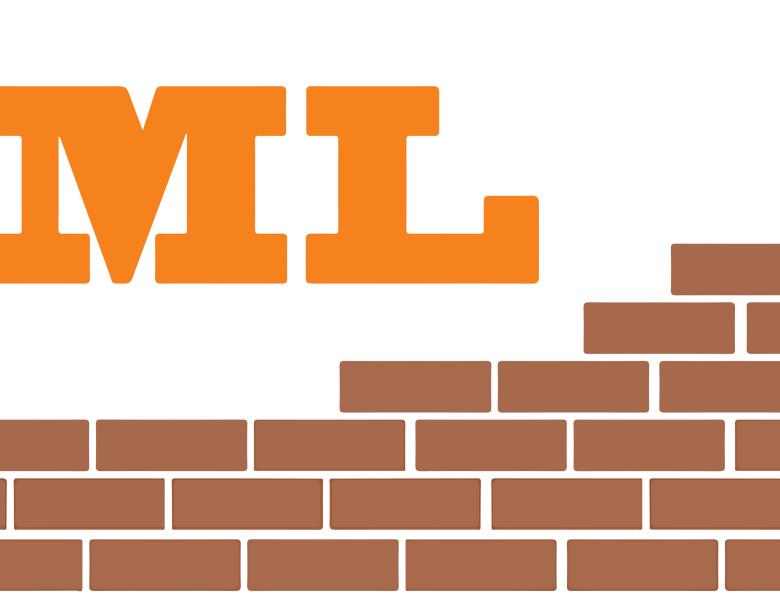
Abstract
The scale and complexity of biological systems makes biological research a fertile domain for active learning. This is because for complex biological systems, time and cost constraints make it infeasible to do all possible experiments. Previous applications of active learning in biology have been limited, and can be divided between retrospective studies, the goal of which is just to demonstrate the usefulness of active learning algorithms, and prospective studies, in which active learning is actually used to drive experimentation. The latter ones are rare. Furthermore, past studies mostly considered unidimensional active learning, where only a single variable is explored (i.e., which member of a set of drugs is most active on a single target). The goal was to reduce cost for a study that would have been feasible but expensive if carried out exhaustively. However, most biological systems have multiple, interacting components, and thus require multidimensional active learning (e.g., choose which pairs of drugs and targets to test in order to model possible effects of multiple drugs on multiple targets). This is far more challenging, but the goal is not just to reduce cost but tackle problems not otherwise addressable. In this talk, I will describe both retrospective and prospective applications of multidimensional active learning to biological systems. Considerations discussed will include the choice of the modeling method, incorporation of prior information using similarity matrices, and how to know when a model is good enough to stop doing experiments.