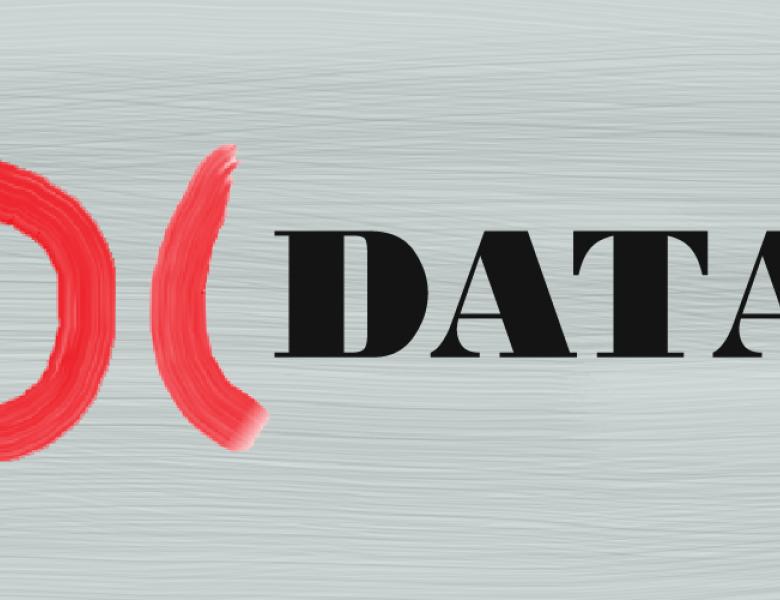
Abstract
Real-time aggregate statistics of data collected from individuals can be shared to enable many applications such as disease surveillance and traffic monitoring. However, it must be ensured that the privacy of individuals is not compromised. While differential privacy has emerged as a de facto standard for private data analysis, directly applying the differential privacy mechanisms on time-series has limited utility due to high correlations between data values. In this talk, I will present FAST, a novel Filtering and Adaptive Sampling based framework for monitoring aggregate Time-series under differential privacy. FAST adaptively samples long time-series according to detected data dynamics and simultaneously uses filtering techniques to dynamically predict and correct released data values. I will present experimental studies using real datasets demonstrating the feasibility and benefit of FAST and conclude with open questions.