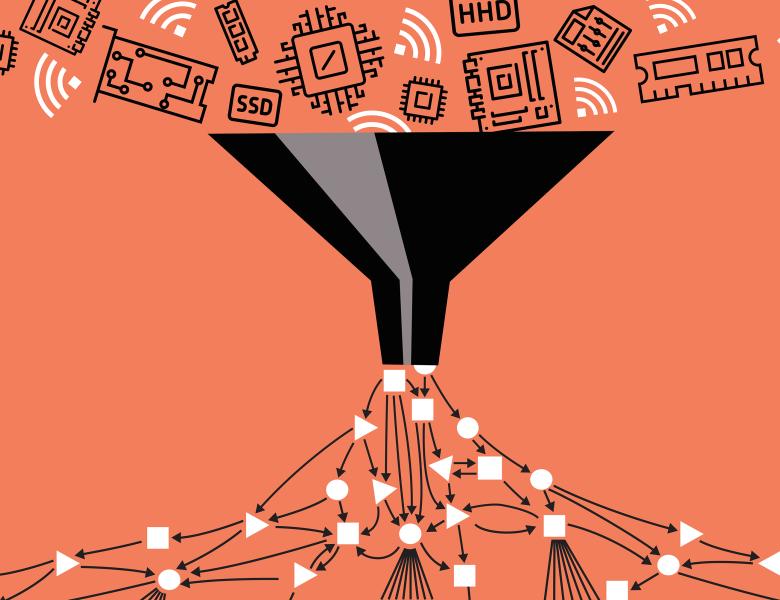
Abstract
The last decade has witnessed tremendous success in using machine learning (ML) in a multitude of safety-critical cyber-physical systems domains thanks to the appeal of designing control systems based on purely data-driven architectures. However, regardless of the explosion in the use of machine learning to design data-driven feedback controllers, providing formal safety and reliability guarantees of these ML-based controllers is in question. In this talk, I will present an approach for the formal training of Neural Network (NN) controllers to enforce formal safety and liveness specifications. Given a nonlinear dynamical model of the system, our approach utilizes ideas from reachability analysis to identify the families of controllers that are guaranteed to satisfy the formal requirements. Next, our approach biases and restricts the training of the NN to those identified controllers. I will discuss several potential applications for such a framework in the context of autonomous cars.