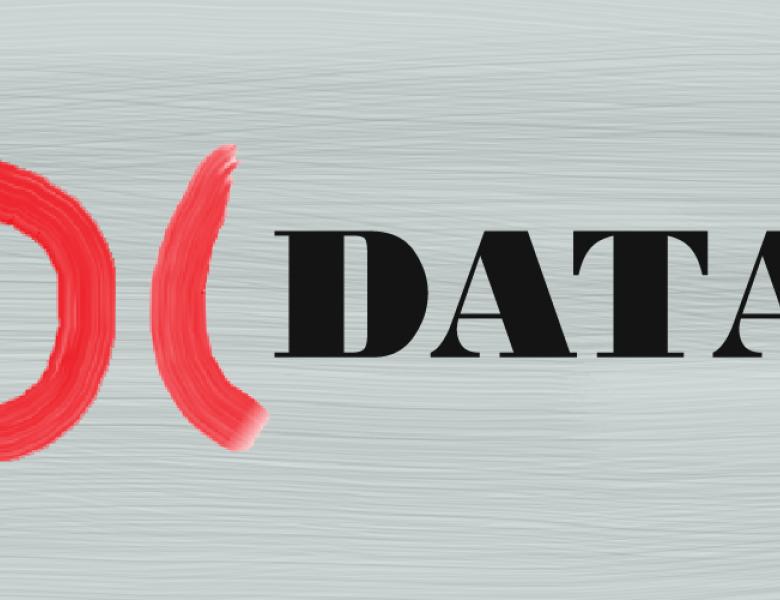
Abstract
We compare the sample complexity of private learning and sanitization tasks under pure eps-differential privacy and approximate (eps, del)-differential privacy. We show that the sample complexity of these tasks under approximate differential privacy can be significantly lower than that under pure differential privacy.
We define a family of optimization problems, which we call Concave Promise Problems, that generalizes some of the tasks we consider. We observe that a concave promise problem can be privately approximated using a solution to a smaller instance of a concave promise problem. This allows us to construct an efficient recursive algorithm solving such problems privately.
Joint work with Amos Beimel and Uri Stemmer.