Image
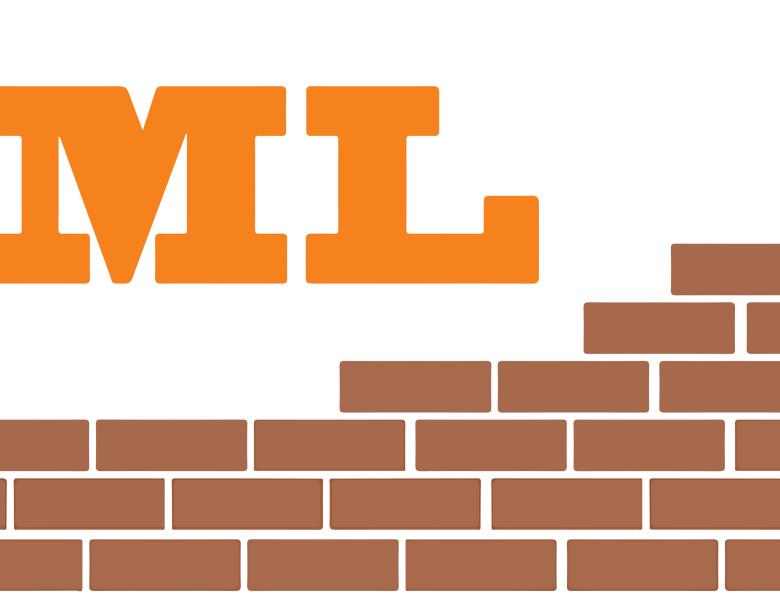
We show that a perturbed form of gradient descent converges to a second-order stationary point in a number iterations which depends only poly-logarithmically on dimension (i.e., it is almost ``dimension-free''). The convergence rate of this procedure matches the well-known convergence rate of gradient descent to first-order stationary points, up to log factors. When all saddle points are non-degenerate, all second-order stationary points are local minima, and our result thus shows that perturbed gradient descent can escape saddle points almost for free.