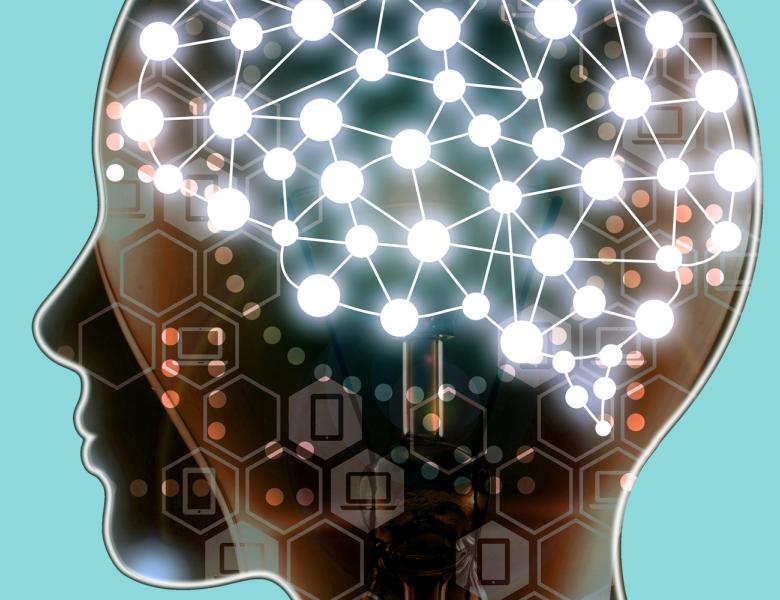
Abstract
This talk will be very informal: I will discuss ongoing research with open questions and partial results.
We study the asymptotic consistency of modern interpolating methods, including deep networks, in both classification and regression settings. That is, we consider learning methods which scale the data size while simultaneously scaling the model size, in a way which always interpolates the train set. We present empirical evidence that, perhaps contrary to intuitions in theory, many natural interpolating learning methods are *inconsistent* for a wide variety of distributions. That is, they do not approach Bayes-optimality even in the limit of infinite data. The message is, in settings with nonzero Bayes risk, overfitting is not benign: interpolating the noise significantly harms the classifier, to the point of preventing consistency.
This work is motivated by: (1) understanding differences between the overparameterized and underparameterized regime, (2) guiding theory towards more "realistic" assumptions to capture deep learning practice, and (3) understanding common structure shared by "natural" interpolating methods.
Based on joint work with: Neil Mallinar, Amirhesam Abedsoltan, Gil Kur, and Misha Belkin. And is a follow-up work to the paper "Distributional Generalization", joint with Yamini Bansal.