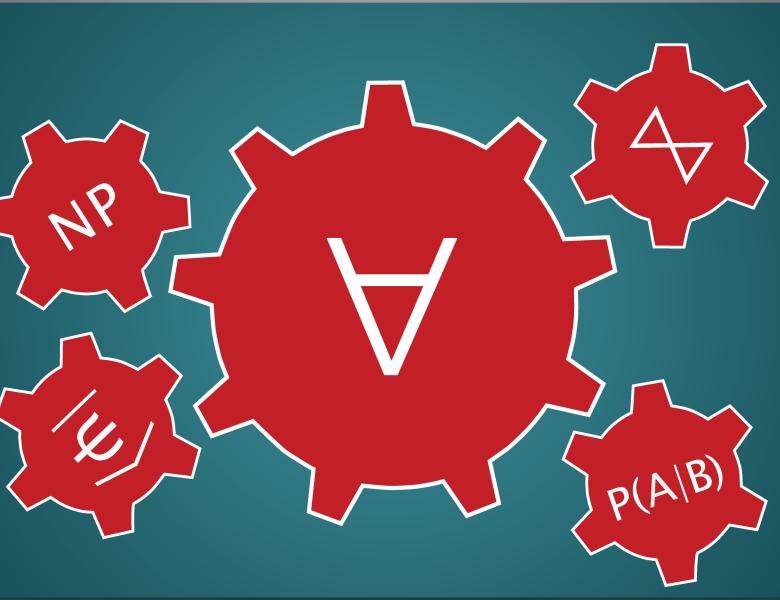
Abstract
In this talk we use a simple auction design problem to illustrate a number of approaches to interpolating between worst- and average-case analysis. Specifically, what selling procedure should a seller use to maximize her revenue? This is a hard question because the best auction to run --- like choosing the right opening bid in an eBay auction or the right reserve price in a sponsored search auction --- depends on what bidders are willing to pay. The straightforward worst-case analysis approach fails to produce any meaningful results. The traditional approach in economics is to maximize the seller's expected revenue with respect to a known distribution over what bidders are willing to pay. Two critiques of this "average-case" approach are: (i) it requires detailed knowledge of the input distribution; and (ii) in many cases, the theoretically optimal auction is too complex for direct use. Are there useful "sweet spots" between worst- and average-case analysis, that inherit the robustness of the former model and the strong guarantees of the latter model, and that naturally lead to plausibly implementable solutions? This talk surveys several such interpolations that have proved useful: partial distributional knowledge, in the form of coarse statistics or independent samples; prior-independent auctions, where the goal is to be simultaneously near-optimal for a wide range of distributions; and using appropriate revenue benchmarks to recover meaningful worst-case guarantees.