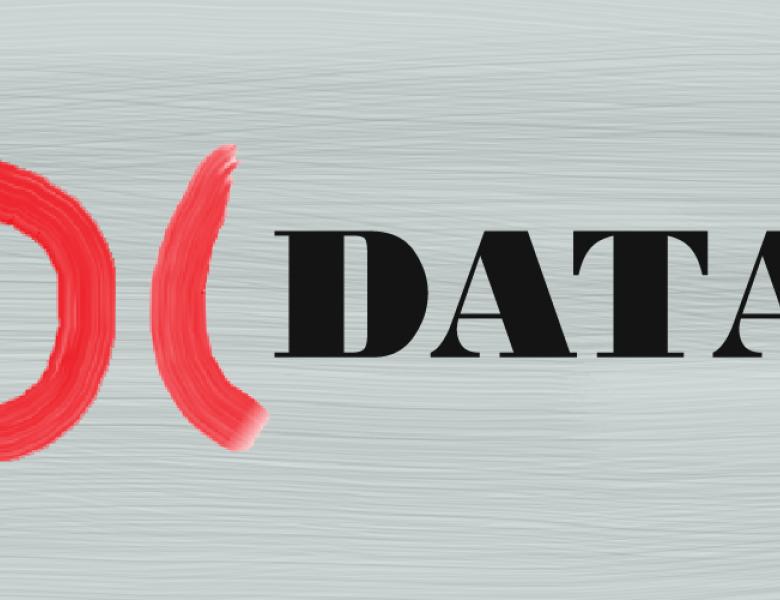
Abstract
Machine learning and computational statistics problems involving large datasets have proved to be a rich source of interesting and challenging optimization problems in recent years. The challenges arising from the complexity of these problems and the special requirements for their solutions have brought a wide range of optimization algorithms into play. We start this talk by surveying the application space, outlining several important analysis and learning tasks, and describing the contexts in which such problems are posed. We then describe optimization approaches that are proving to be relevant, including stochastic gradient methods, sparse optimization methods, first-order methods, coordinate descent, higher-order methods, and augmented Lagrangian methods. We also discuss parallel variants of some of these approaches.
The first session of this talk will take place on Wednesday, September 4 from 4:00 pm – 5:30 pm.