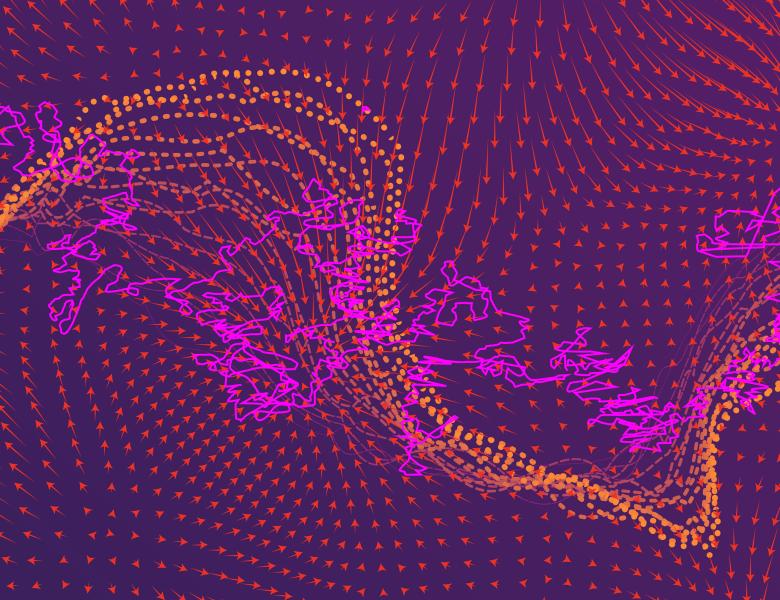
Abstract
Over the past ten years, optimal transport has become a fundamental tool in statistics and machine learning: the Wasserstein metric provides a new notion of distance for classifying distributions and a rich geometry for interpolating between them. In parallel, optimal transport has gained mathematical significance by providing new tools for studying stability and long time behavior of partial differential equations, through the theory of Wasserstein gradient flows. These two lines of research have recently intersected in work on sampling and the dynamics of training neural networks with a single hidden layer. In this talk, I will introduce the theory of Wasserstein gradient flows, focusing on the interplay between convexity of an energy, curvature of the metric space, and dynamics of gradient flows.