Image
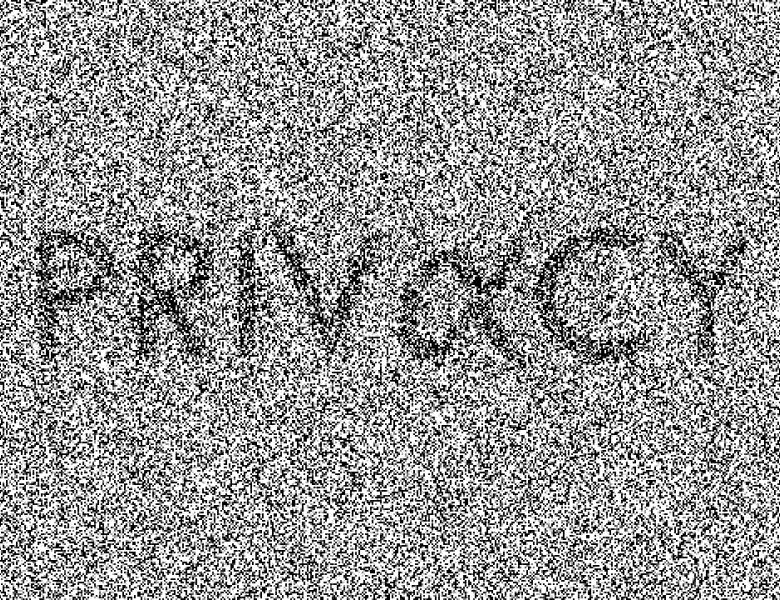
We propose a Bayesian approach to measure loss of privacy and apply it to the design of optimal mechanisms. The privacy loss associated with a mechanism is defined as the difference between the designer's prior and posterior beliefs about an agent's type, where this difference is calculated using Kullback-Leibler divergence, and where the change in beliefs is triggered by the agent's actions in the mechanism. We consider both ex-ante and ex-post (with respect to the type realizations) measures of privacy loss and apply them to study the properties of optimal privacy-constrained mechanisms and the relation between welfare/profits and privacy levels.