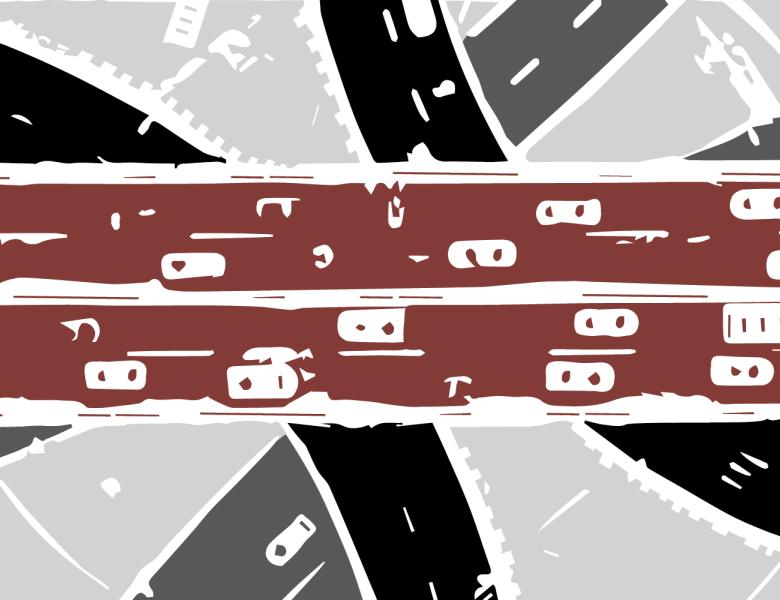
Abstract
The NOAA Warn-on-Forecast (WoF) program is developing thunderstorm-scale (1-3-km grid spacing) numerical weather prediction (NWP) systems that will be used by National Weather Service (NWS) forecasters to improve 0-3-h forecasts of tornadoes, large hail, damaging wind, and flash flooding. Due to the inherent limitations of atmospheric observations and current NWP models, storm-scale forecasts are characterized by large uncertainty. Storm-scale NWP systems therefore use an ensemble of O(10) members to provide probabilistic forecast information. Ensemble analyses of storms and the larger-scale environment are generated by assimilating radar, satellite, surface, and upper-air observations every 5-15 min into the three-dimensional model system state (e.g., wind, temperature, humidity, precipitation). Data assimilation at these scales typically uses one of two approaches. The most common is the ensemble Kalman filter (EnKF), which uses statistics from the (non-linear) ensemble forecasts to estimate the covariances between observations and the system state vector to update the analysis. Another common approach uses a hybrid framework that leverages both the flow-dependent covariances provided by the EnKF and the dynamical constraints (e.g., mass conservation) provided by (fixed-covariance) variational approaches. The resulting ensemble of analyses characterizes the uncertainty in the initial atmospheric state. Varying the physics parameterization schemes among the ensemble members additionally accounts for the uncertainty in the model. Thus, the ensemble forecast initialized from the ensemble analysis ideally provides a representative sampling of the probability distribution function of the future state of the atmosphere.
The ensemble prediction framework presents significant challenges, however. First, large forecast errors still frequently occur, and these arise partly from computational limitations imposed by the real-time requirement. For example, the NWP models cannot be run at arbitrarily fine resolution if forecasts are to be available well in advance of the event; ensemble membership sizes are likewise limited. This has motivated a series of predictability studies to evaluate the impacts of different forecast error sources. These studies will provide valuable guidance for optimizing the design of WoF systems. In addition, initial efforts are underway to apply machine learning techniques (e.g., random forests) to ensemble forecast output to mitigate the impacts of model errors.
A second major challenge is the massive data volume generated by storm-scale ensembles. Each forecast typically comprises O(10) ensemble members, O(10) prognostic and diagnostic variables, O(1,000,000) grid points, and O(10) forecast output times. Due to the rapid evolution of severe thunderstorms, forecasters do not have time to thoroughly interrogate all of this output. This requires that ensemble forecasts be post-processed and distilled into the most operationally relevant severe thunderstorm guidance. Using output from a prototype WoF prediction system that is run each spring, physical and social scientists have begun working closely with NWS forecasters to learn how to tailor the forecast guidance to their needs. The guidance is made available in real-time on a public web page, which poses an additional computational challenge.