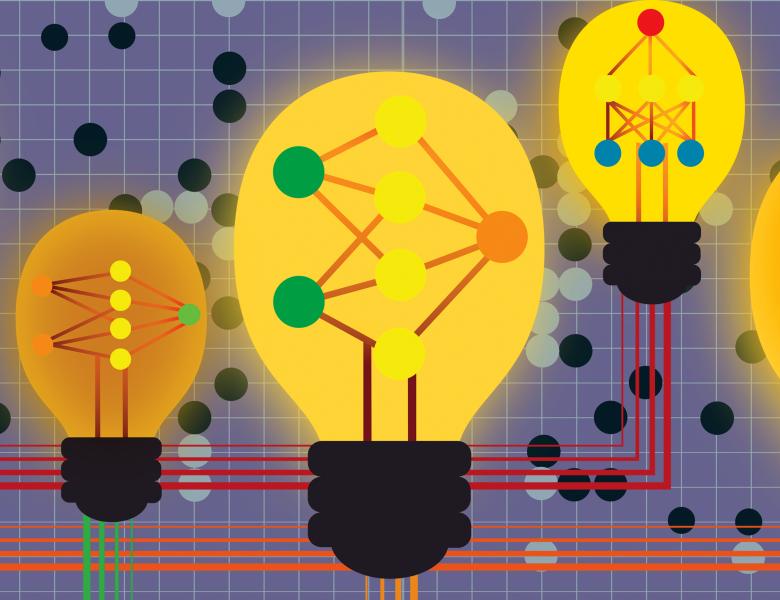
Abstract
I will discuss some recent results on learning approximate Nash equilibrium policies in nonzero-sum stochastic dynamic games using the framework of mean-field games (MFGs). Following a general introduction, I will focus, for concrete results, on the structured setting of discrete-time infinite-horizon linear-quadratic-Gaussian dynamic games, where the players (agents) are partitioned into finitely-many populations connected by a network of known structure. Each population has a high number of agents, which are indistinguishable, but there is no indistinguishability across different populations. It is possible to characterize the Nash equilibrium (NE) of the game when the number of agents in each population goes to infinity, the so-called mean-field equilibrium (MFE), with local state information for each agent (thus making scalability not an issue), which can then be shown to lead to an approximate NE when the population sizes are finite, with a precise quantification of the approximation as a function of population sizes. The main focus of the talk, however, will be the model-free versions of such games, for which I will introduce a learning algorithm, based on zero-order stochastic optimization, for computation of the MFE, along with guaranteed convergence. The algorithm exploits the affine structure of both the equilibrium controller (for each population) and the equilibrium MF trajectory by decomposing the learning task into learning first the linear terms, and then the affine terms. One can also obtain a finite-sample bound quantifying the estimation error as a function of the number of samples. The talk will conclude with discussion of some extensions of the setting and future research directions.