Image
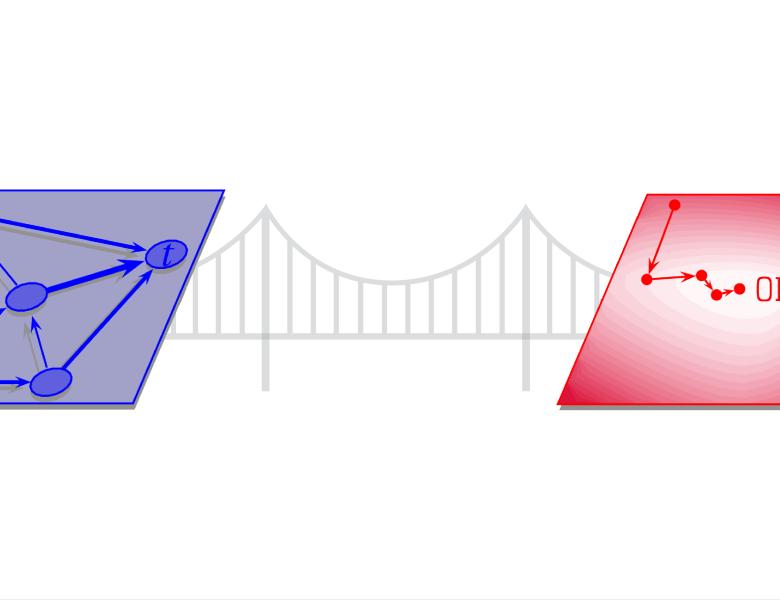
Much empirical work in deep learning has gone into avoiding vanishing gradients, a necessary condition for the success of stochastic gradient methods. This raises the question of whether we can provably rule out vanishing gradients for some expressive model architectures? I will point out several obstacles, as well as positive results for some simplified architectures, specifically, linearized residual networks, and linear dynamical systems.
Based on joint works with Ma and Recht.