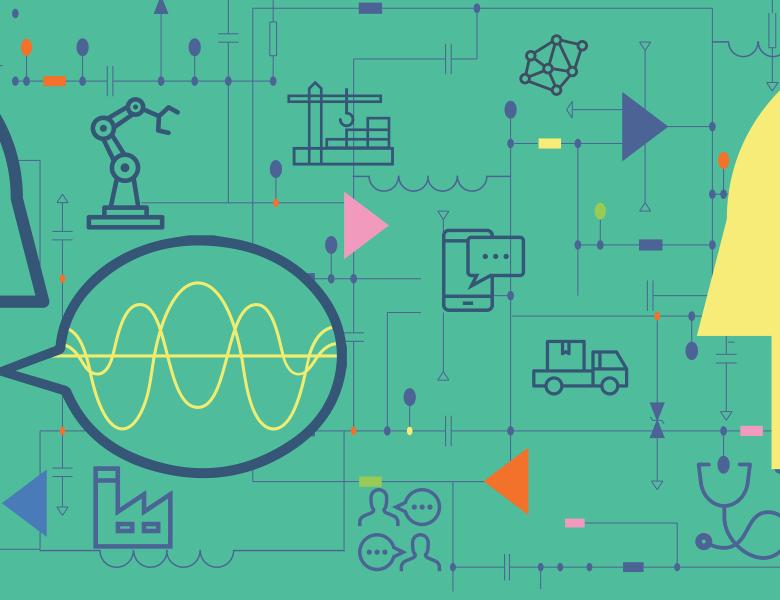
Abstract
Offline reinforcement learning (RL) refers to the problem of learning policies entirely from a batch of previously collected data. In this talk, I will discuss MOPO, a model-based offline deep reinforcement learning algorithm. The gist is to modify existing model-based RL methods by introducing a penalty on the reward based on the uncertainty quantification of the learned dynamics. We maximize the penalized return, which is theoretically shown to be a lower bound for the true MDP's return. MOPO outperforms standard model-based RL methods and existing state-of-the-art model-free offline RL approaches on offline RL benchmarks.