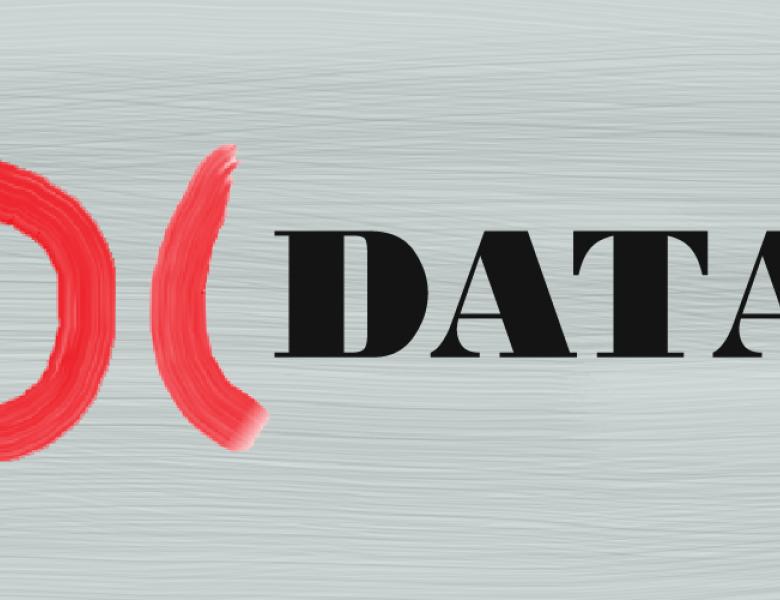
Abstract
Graphical models are a popular tool for understanding dependency structure of multivariate data, and sparse graphical models can provide an informative and interpretative summary of high-dimensional data. The commonly used graphical models are usually one of two types: Gaussian graphical models (for continuous data) and the Ising models or Markov networks (for binary and discrete data). However, in practice both types of variables are frequently present in the same dataset, creating the need for mixed graphical models. Some models for these were developed in the earlier literature, but none of them scale to high dimensions. We propose a novel graphical model for mixed data, which is simple enough to be suitable for high-dimensional data, yet flexible enough to represent all possible graph structures, and develop a computationally efficient algorithm for fitting the model. We will also discuss another extension of the graphical model that allows the graph to depend on additional covariates, and apply it to data on genetic instability in tumor samples.