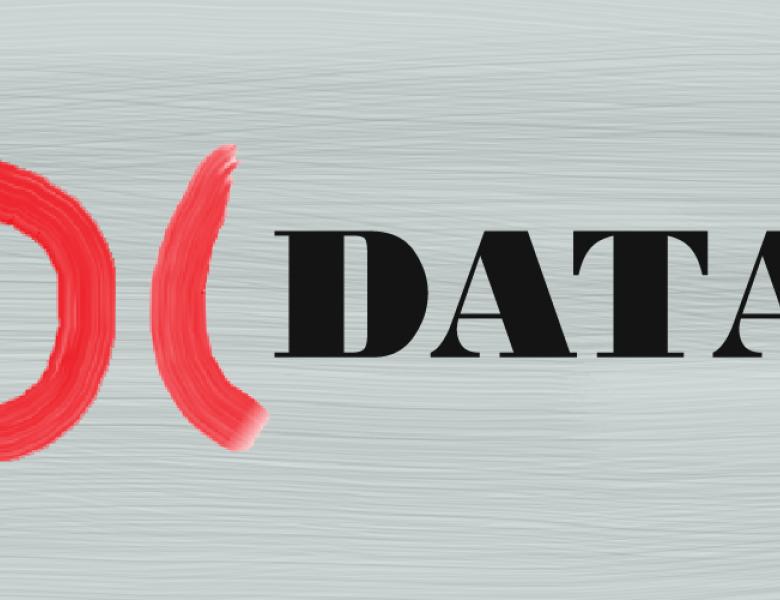
Abstract
The rapid growth in the size and scope of datasets in science and technology has created a need for novel foundational perspectives on data analysis that blend the computational and statistical sciences. That classical perspectives from these fields are not adequate to address emerging problems in "Big Data" is apparent from their sharply divergent nature at an elementary level—in computer science, the growth of the number of data points is a source of "complexity" that must be tamed via algorithms or hardware, whereas in statistics, the growth of the number of data points is a source of "simplicity" in that inferences are generally stronger and asymptotic results can be invoked. Indeed, if data are a statistician's principal resource, why should more data be burdensome in some sense? Shouldn't it be possible to exploit the increasing inferential strength of data at scale to keep computational complexity at bay? I present several research vignettes that attempt to bring together computational and statistical thinking.
Related Links
A scalable bootstrap for massive data. A. Kleiner, A. Talwalkar, P. Sarkar and M. I. Jordan. Journal of the Royal Statistical Society, Series B, in press.
arxiv.org/abs/1112.5016
On statistics, computation and scalability. M. I. Jordan. Bernoulli, 19, 1378-1390, 2013.
www.cs.berkeley.edu/~
Computational and statistical tradeoffs via convex relaxation. V. Chandrasekaran and M. I. Jordan. Proceedings of the National Academy of Sciences, 110, E1181-E1190, 2013.
www.pnas.org/content/