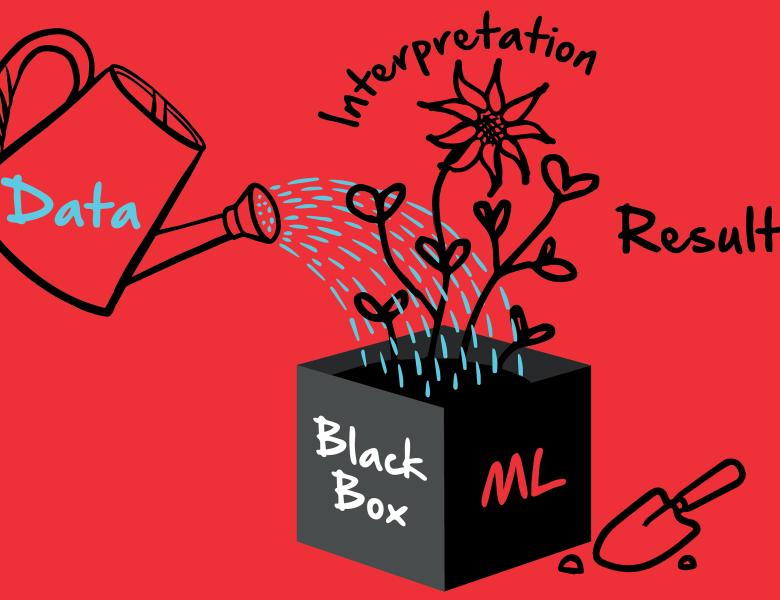
Abstract
The mammalian brain is an extremely complicated, dynamical deep network. Systems, cognitive and computational neuroscientists seek to understand how information is represented throughout this network, and how these representations are modulated by attention and learning. Machine learning provides many tools useful for analyzing brain data recorded in neuroimaging, neurophysiology and optical imaging experiments. For example, deep neural networks trained to perform complex tasks can be used as a source of features for data analysis, or they can be trained directly to model complex data sets. Although artificial deep networks can produce complex models that accurately predict brain responses under complex conditions, the resulting models are notoriously difficult to interpret. This limits the utility of deep networks for neuroscience, where interpretation is often prized over absolute prediction accuracy. In this talk I will review two approaches that can be used to maximize interpretability of artificial deep networks and other machine learning tools when applied to brain data. The first approach is to use deep networks as a source of features for regression-based modeling. The second is to use deep learning infrastructure to construct sophisticated computational models of brain data. Both these approaches provide a means to produce high-dimensional quantitative models of brain data recorded under complex naturalistic conditions, while maximizing interpretability.