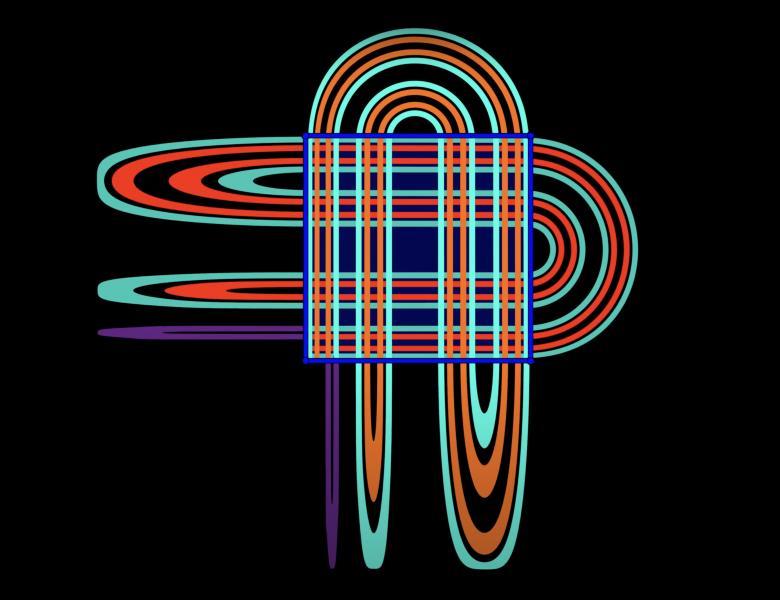
Abstract
My talk reveals the potassium resistance R and sodium resistance RKNa shown in Figure 1 of the monumental 1952 paper which Hodgkin and Huxley had mis- identified as time-varying resistances , are erroneous , and must be reclassified as memristors , the fourth basic electrical circuit element . It has since been identified as synapses in neuromorphic system models, and as future workhorse for model parameter learning and massive AI computing. From a foundational perspective , I will use the memristor to resolve 4 heretofore unsolved classic problems in neuroscience , namely, the Galvani's irritability , dating back 243 years, the classic "all-or-none" mystery , the Turing Instability , and the Smale Paradox. My resolution of these unresolved problems was made possible by exploiting the Principle of local activity , which , within a certain relatively small parameter region, could harbour a physical state , dubbed edge of chaos. In my talk , I will provide the explicit formula for calculating, via matrix algebra , the precise parameter range where a nonlinear system is locally active , orenturing deeper into an often miniscule domain of edge of chaos . Unlike numerous unsuccessful attempts , such as Boltzmann's assay for decreasing entropy, Schrodonger's futile search for negative entropy, Prigogine's quest for the "instability of the
homogeneous", and Gell-Mann's musing on "amplification of fluctuations" , the principle of local activity provides an explicit formula to identify the parameter nook where edge of chaos reigns supreme.