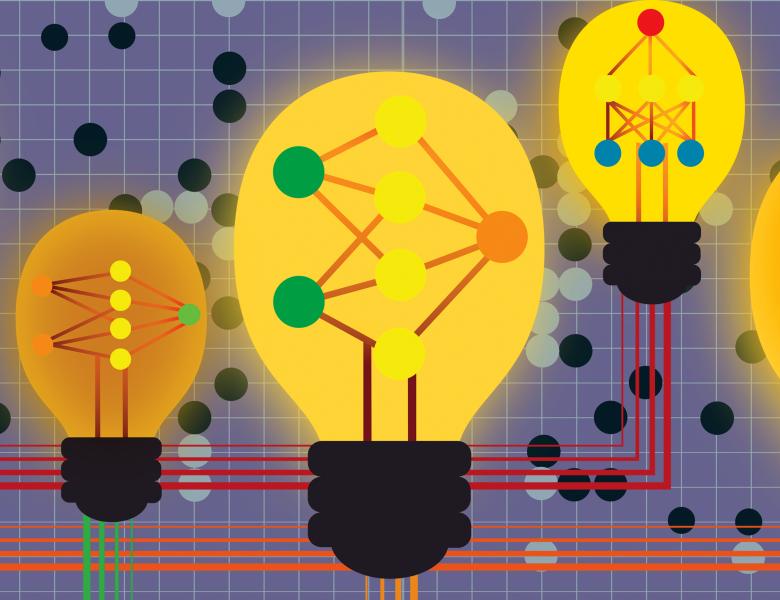
Abstract
In many learning scenarios an important aim is to learn a representation that does not carry any information about some feature of the input. For privacy reasons, the identity of an input datum should be obfuscated; for fairness considerations, surfacing particular attributes may be undesirable; and disentanglement entails separating the learned subspaces of the representation. In this talk I will discuss methods for accomplishing this, and the degree to which they have been successful.