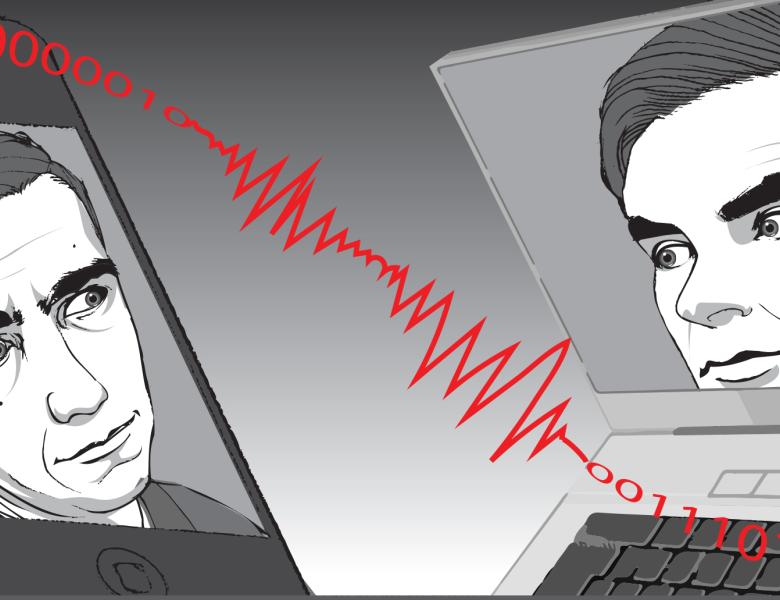
Abstract
I will focus on a core problem in statistical estimation: how to infer information about a distribution based on random samples. I will describe an algorithmic framework that yields new, provably efficient estimators for several natural and well-studied statistical models, including mixtures of structured distribution families (e.g., gaussian, log-concave, etc.). This framework provides a fairly complete picture of the sample and computational complexities for fundamental inference tasks, including density estimation and hypothesis testing.