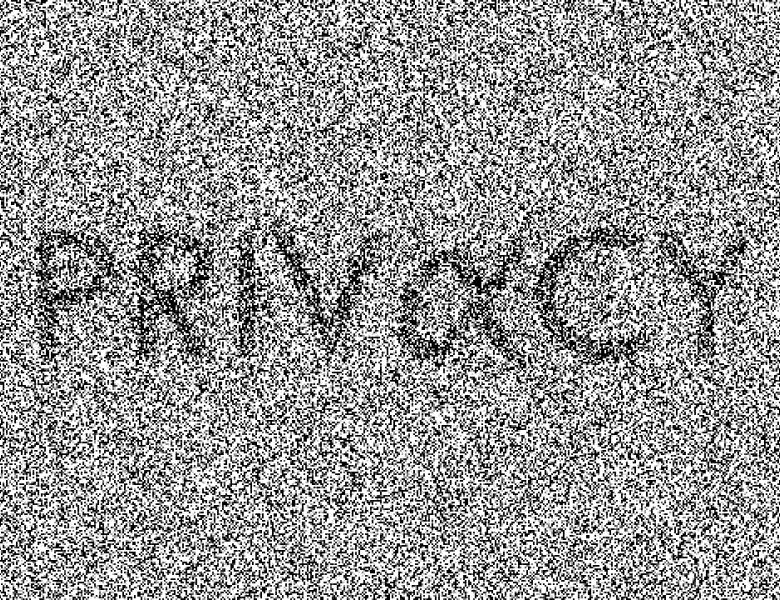
Abstract
Differential privacy is a mathematical framework for privacy-preserving data analysis. Changing the hyper-parameters of a differentially private algorithm allows one to trade off privacy and utility in a principled way. Quantifying such trade-off in advance is essential to decision-makers tasked with deciding how much privacy can be provided in a particular application while keeping acceptable utility. Analytical utility guarantees offer a simple tool to reason about this trade-off, but they are generally only available for relatively simple problems. For more complex tasks, including the training of neural networks under differential privacy, the utility achieved by a given algorithm can only be measured empirically. This paper presents a Bayesian optimization methodology for efficiently characterizing the privacy-utility trade-off of any differentially private algorithm using only empirical measurements of its utility. The versatility of our methods is illustrated on a number of machine learning tasks involving multiple models, optimizers, and datasets.